Recognition Method of Highway Geological Hazards Based on Pre-trained DCNN
A technology of geological disasters and recognition methods, applied in character and pattern recognition, computer parts, instruments, etc., can solve problems such as low interpretation efficiency, reduce personal safety risks, and improve efficiency
- Summary
- Abstract
- Description
- Claims
- Application Information
AI Technical Summary
Problems solved by technology
Method used
Image
Examples
Embodiment Construction
[0050] The present invention will be further described in detail below in conjunction with the accompanying drawings and specific embodiments.
[0051] In recent years, with the rapid development of computer technology, deep learning has been widely used in many fields such as computer vision, speech recognition and natural language processing. Convolutional Neural Networks (CNN, Convolutional Neural Networks) is currently the most studied and most mature model in deep learning. It has been successfully applied in high-resolution remote sensing image classification, feature extraction, and scene recognition. However, CNN model training requires the use of a large number of labeled samples. Only when the number of training samples is large enough and the network structure is relatively complex can it show excellent performance; and in the absence of training samples, the network is prone to overfitting. The combination and fall into the local optimal solution and so on.
[005...
PUM
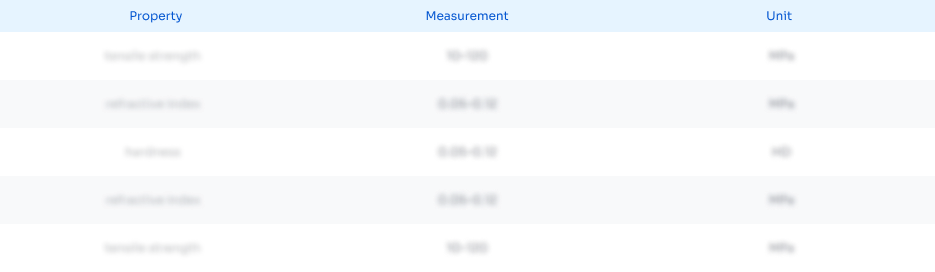
Abstract
Description
Claims
Application Information
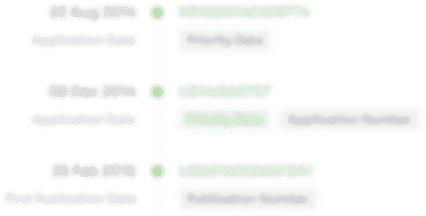
- R&D Engineer
- R&D Manager
- IP Professional
- Industry Leading Data Capabilities
- Powerful AI technology
- Patent DNA Extraction
Browse by: Latest US Patents, China's latest patents, Technical Efficacy Thesaurus, Application Domain, Technology Topic, Popular Technical Reports.
© 2024 PatSnap. All rights reserved.Legal|Privacy policy|Modern Slavery Act Transparency Statement|Sitemap|About US| Contact US: help@patsnap.com