Pedestrian re-identification method based on transfer learning and deep feature fusion
A pedestrian re-identification and deep feature technology, applied in neural learning methods, character and pattern recognition, instruments, etc., can solve the problem of not considering the difference in data distribution, not fully utilizing the deep fusion of pedestrian local features, and the network migration effect is not ideal, etc. problems, to achieve strong resolution and improve accuracy
- Summary
- Abstract
- Description
- Claims
- Application Information
AI Technical Summary
Problems solved by technology
Method used
Image
Examples
Embodiment 1
[0080] As mentioned above, a pedestrian re-identification method based on transfer learning and deep feature fusion includes the following steps:
[0081] ① Pre-training: Pre-training the pre-training model based on ImageNet on the pedestrian re-recognition data to obtain the pedestrian re-recognition pre-training network model; specifically divided into the following steps:
[0082] (1.1) Obtain the pre-trained deep convolutional network model on the ImageNet data set, and train it on the pedestrian re-recognition data;
[0083] (1.2) When pre-training the deep convolutional neural network model on pedestrian re-identification data, only the sample annotation information is used to fine-tune the deep convolutional network model;
[0084] (1.2.1) The ResNet50 network model pre-trained on the ImageNet dataset will remove the top fully connected layer, and add two fully connected layers and a softmax layer after the maximum pooling layer;
[0085] (1.2.2) Use the tag information of the pe...
Embodiment 2
[0132] As mentioned above, a pedestrian re-identification method based on transfer learning and deep feature fusion includes the following steps:
[0133] Step S1, pre-training the pre-training model based on ImageNet on the pedestrian re-recognition data to obtain the pedestrian re-recognition pre-training network model;
[0134] Step S11: Obtain a pre-trained deep convolutional network model on the ImageNet data set, and train it on the pedestrian re-recognition data;
[0135] Step S12, when the deep convolutional neural network model is pre-trained on the pedestrian re-identification data, only the sample annotation information is used to fine-tune the network model;
[0136] Step S121, removing the fully connected layer at the top of the ResNet50 network model pre-trained on the ImageNet dataset, and adding two fully connected layers and a softmax layer after the maximum pooling layer;
[0137] Furthermore, the parameters of the two fully connected layers added are 1×1×2048, 1×1×751...
PUM
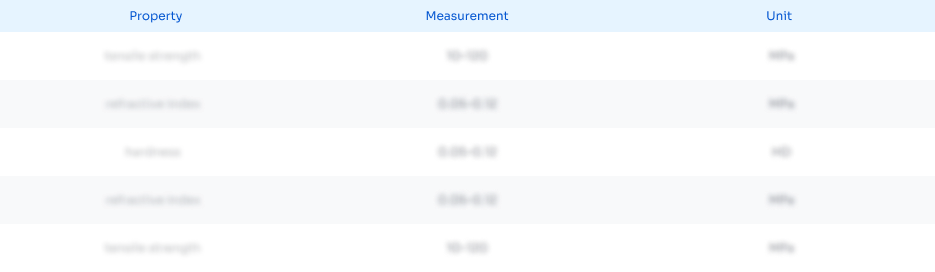
Abstract
Description
Claims
Application Information
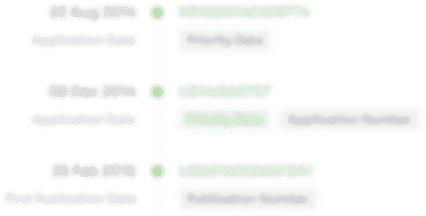
- R&D Engineer
- R&D Manager
- IP Professional
- Industry Leading Data Capabilities
- Powerful AI technology
- Patent DNA Extraction
Browse by: Latest US Patents, China's latest patents, Technical Efficacy Thesaurus, Application Domain, Technology Topic.
© 2024 PatSnap. All rights reserved.Legal|Privacy policy|Modern Slavery Act Transparency Statement|Sitemap