Universal no-reference image quality evaluation method based on multi-task convolutional neural network
A convolutional neural network, reference image technology, applied in the field of general non-reference image quality evaluation, to achieve the effect of accurate image representation and good performance
- Summary
- Abstract
- Description
- Claims
- Application Information
AI Technical Summary
Problems solved by technology
Method used
Image
Examples
Embodiment Construction
[0041] In order to make the object, technical solution and advantages of the present invention clearer, the present invention will be further described in detail below in conjunction with the accompanying drawings and embodiments. It should be understood that the specific embodiments described here are only used to explain the present invention, and do not limit the protection scope of the present invention.
[0042] Such as figure 1 As shown, the non-reference image quality evaluation method based on the multi-task convolutional neural network provided in this embodiment includes the following steps:
[0043] Step 1: Build training data
[0044] The data set used for training the multi-task neural network proposed by the present invention is a LIVE data set.
[0045] The LIVE dataset has 29 original images and 792 corresponding degraded images. The degraded categories include five categories: JPEG, JPEG2000, Noise, Blur and Fast Fading. Each contaminated image has a human-...
PUM
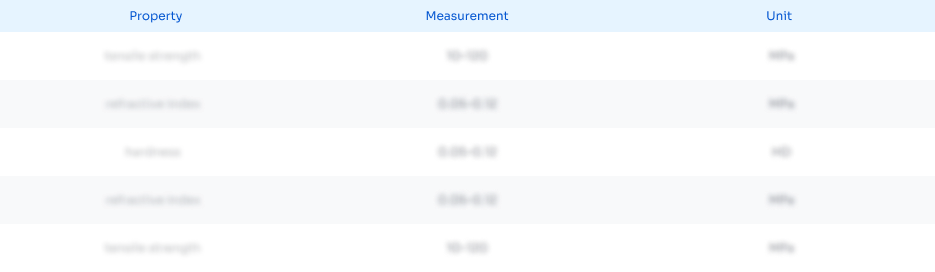
Abstract
Description
Claims
Application Information
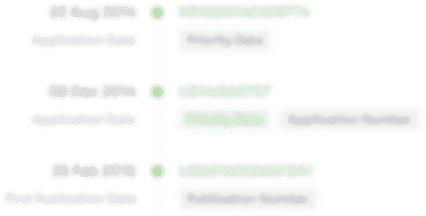
- R&D Engineer
- R&D Manager
- IP Professional
- Industry Leading Data Capabilities
- Powerful AI technology
- Patent DNA Extraction
Browse by: Latest US Patents, China's latest patents, Technical Efficacy Thesaurus, Application Domain, Technology Topic, Popular Technical Reports.
© 2024 PatSnap. All rights reserved.Legal|Privacy policy|Modern Slavery Act Transparency Statement|Sitemap|About US| Contact US: help@patsnap.com