Image scene labeling method based on conditional random field and secondary dictionary study
A conditional random field and dictionary learning technology, which is applied to computer parts, character and pattern recognition, instruments, etc., can solve the problems that affect the correctness of target classification and cannot distinguish which category of dictionary primitives, and achieve consistency preservation, The effect of improving accuracy
- Summary
- Abstract
- Description
- Claims
- Application Information
AI Technical Summary
Problems solved by technology
Method used
Image
Examples
Embodiment Construction
[0032] Below in conjunction with accompanying drawing, technical scheme of the present invention is described in further detail:
[0033] Such as figure 1 As shown, the present invention designs a scene semantic annotation framework based on conditional random field and secondary dictionary learning. In the actual application process, the basic second-order CRF semantic annotation framework is used, and the histogram composed of sparse codes based on dictionary learning Semantic annotation of scene images as a high-level item of CRF extension. The semantic annotation framework is composed of second-order potential energy composed of bottom-up regional level and high-order potential energy composed of top-down category-level information, including the following steps:
[0034] Step A. Perform superpixel over-segmentation on the training set images, and obtain the superpixel over-segmentation region of each image;
[0035] Step A1. For each pixel, draw a circle with its locati...
PUM
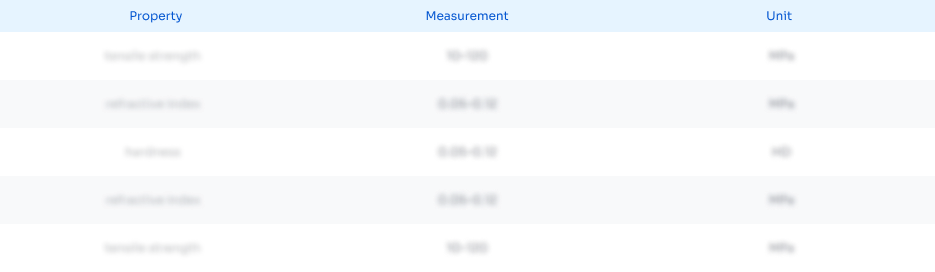
Abstract
Description
Claims
Application Information
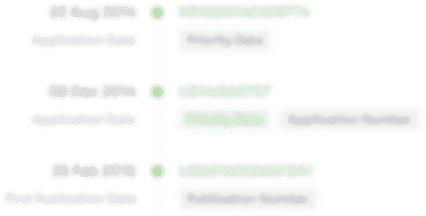
- R&D
- Intellectual Property
- Life Sciences
- Materials
- Tech Scout
- Unparalleled Data Quality
- Higher Quality Content
- 60% Fewer Hallucinations
Browse by: Latest US Patents, China's latest patents, Technical Efficacy Thesaurus, Application Domain, Technology Topic, Popular Technical Reports.
© 2025 PatSnap. All rights reserved.Legal|Privacy policy|Modern Slavery Act Transparency Statement|Sitemap|About US| Contact US: help@patsnap.com