Double-energy-spectrum CT projection domain base material decomposition method and device based on deep learning
A deep learning and base material technology, applied in neural learning methods, projection reproduction, 2D image generation, etc., can solve problems such as limited application scope, and achieve simple training phantoms, strong flexibility, and good anti-noise performance. Effect
- Summary
- Abstract
- Description
- Claims
- Application Information
AI Technical Summary
Problems solved by technology
Method used
Image
Examples
Embodiment Construction
[0054] The following will clearly and completely describe the technical solutions in the embodiments of the present invention with reference to the accompanying drawings in the embodiments of the present invention. Obviously, the described embodiments are only some of the embodiments of the present invention, not all of them. Based on the embodiments of the present invention, all other embodiments obtained by persons of ordinary skill in the art without making creative efforts belong to the protection scope of the present invention.
[0055] The polychromatic projection formula measured after the X-ray passes through the object along a ray L is as follows:
[0056]
[0057] where q m (L) is the X-ray energy spectrum w normalized by the mth m (E) When scanning the measured object, the detected polychromatic projection; μ(x, E) is the linear attenuation coefficient of the measured object at point x with respect to energy E; S m is the influence of scattering and noise detec...
PUM
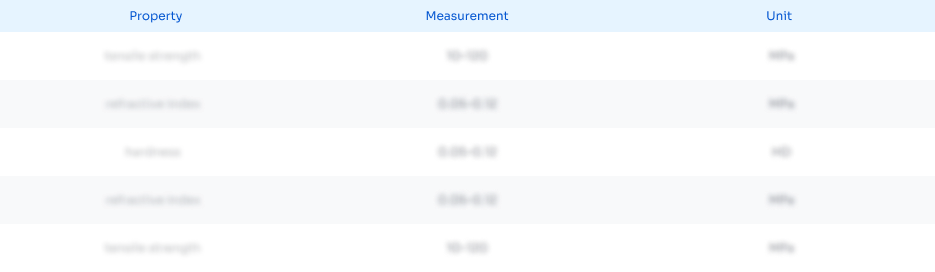
Abstract
Description
Claims
Application Information
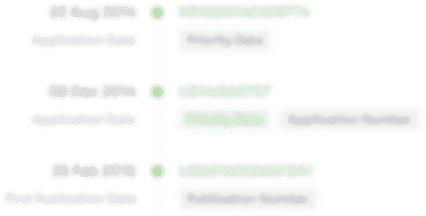
- R&D Engineer
- R&D Manager
- IP Professional
- Industry Leading Data Capabilities
- Powerful AI technology
- Patent DNA Extraction
Browse by: Latest US Patents, China's latest patents, Technical Efficacy Thesaurus, Application Domain, Technology Topic, Popular Technical Reports.
© 2024 PatSnap. All rights reserved.Legal|Privacy policy|Modern Slavery Act Transparency Statement|Sitemap|About US| Contact US: help@patsnap.com