Fast light super-resolution reconstruction dense residual error network
A super-resolution reconstruction and network technology, applied in biological neural network models, image data processing, instruments, etc., can solve problems such as gradient explosion, disappearance, noise, etc., to avoid the introduction of new noise and easy to train.
- Summary
- Abstract
- Description
- Claims
- Application Information
AI Technical Summary
Problems solved by technology
Method used
Image
Examples
Embodiment Construction
[0036] The fast and light super-resolution reconstruction dense residual network is divided into two channels, and the shallow channel only contains 3 convolutional layers and a deconvolutional layer to restore the external contour information of the image. The main task of reconstructing HR images is done by deep channels. The deep channel consists of four parts: feature extraction, nonlinear mapping, upsampling, and multi-scale reconstruction. The specific implementation process of the network will be introduced in detail below.
[0037] 1 data set
[0038] 1.1 Training Dataset
[0039] Use General-100, Yang et al.'s 91image and 200 images of the Berkeley Segmentation Dataset (BSD) as training data. The General-100 dataset contains 100 uncompressed images in bmp format; 91image contains 91 images, so The original data set has a total of 391 pictures. Usually, the larger the data, the better the training effect. In order to make full use of the training data, we use 3 data...
PUM
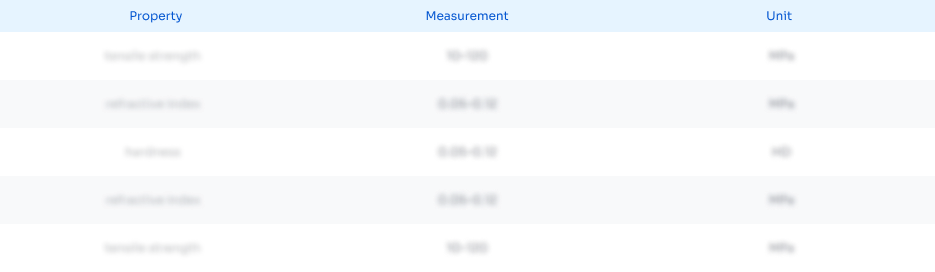
Abstract
Description
Claims
Application Information
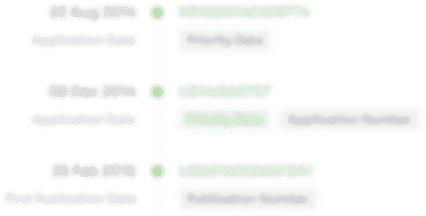
- R&D Engineer
- R&D Manager
- IP Professional
- Industry Leading Data Capabilities
- Powerful AI technology
- Patent DNA Extraction
Browse by: Latest US Patents, China's latest patents, Technical Efficacy Thesaurus, Application Domain, Technology Topic.
© 2024 PatSnap. All rights reserved.Legal|Privacy policy|Modern Slavery Act Transparency Statement|Sitemap