Multi-label image retrieval method fusing triple loss and generative adversarial network
An image retrieval, multi-label technology, applied in metadata still image retrieval, still image data retrieval, biological neural network model, etc., to achieve the effect of expanding the amount of training data and improving retrieval speed and accuracy
- Summary
- Abstract
- Description
- Claims
- Application Information
AI Technical Summary
Problems solved by technology
Method used
Image
Examples
Embodiment
[0076] like figure 1 As shown, an embodiment of the present invention provides a multi-label image retrieval method that combines triplet loss and generative confrontation network, including steps S1-S6.
[0077] Step S1: Build a deep learning framework, deploy a generative adversarial network model, and the generative adversarial network model includes a deep hash coding network.
[0078] S1-1. Build the Caffe deep learning open source framework, and deploy the DCGAN model in the Caffe deep learning open source framework.
[0079] Specifically, in step S1, the present invention builds a Caffe (Convolutional Architecture for Fast Feature Embedding, convolutional architecture for fast feature embedding) deep learning framework. In this embodiment, DCGAN (Deep convolutional generative adversarial networks) network structure (for example, VGG16 can be used) is used as the image generation model. Take the original loss function of the GAN model as its loss function and optimizat...
PUM
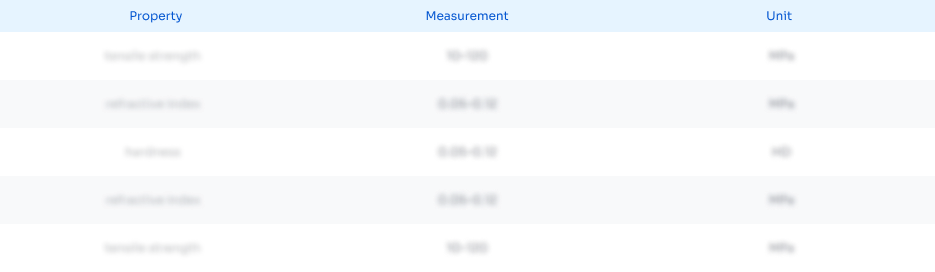
Abstract
Description
Claims
Application Information
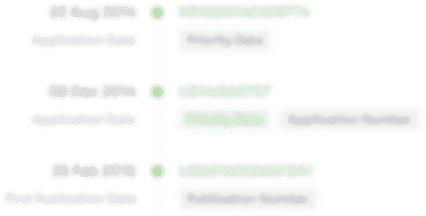
- R&D Engineer
- R&D Manager
- IP Professional
- Industry Leading Data Capabilities
- Powerful AI technology
- Patent DNA Extraction
Browse by: Latest US Patents, China's latest patents, Technical Efficacy Thesaurus, Application Domain, Technology Topic.
© 2024 PatSnap. All rights reserved.Legal|Privacy policy|Modern Slavery Act Transparency Statement|Sitemap