Audio representation learning method based on multilayer time sequence pooling
A learning method and time sequence technology, applied in audio data retrieval, audio data clustering/classification, speech analysis, etc., can solve problems such as the lack of flexible and efficient capture of time series dynamic information, and achieve the effect of improving performance and improving performance.
- Summary
- Abstract
- Description
- Claims
- Application Information
AI Technical Summary
Problems solved by technology
Method used
Image
Examples
specific Embodiment approach 1
[0019] Specific implementation mode one: combine figure 1 This embodiment is described. The audio representation learning method based on multi-layer temporal pooling given in this embodiment specifically includes the following steps:
[0020] Step 1. Extract the spectral features of each audio sample in the training set and the audio to be represented, and divide each spectral feature into segments of equal length, so as to obtain the segment-level time-frequency feature set of the training set and the segment-level of the audio to be represented Time-frequency feature set, using the segment-level time-frequency feature set of the training set to train the CNN network (the time-frequency feature set is used as the input of the CNN network, and the CNN network outputs its representation vector for each segment-level time-frequency feature), and then the training A good CNN network acts as a segment-level feature representation extractor to extract the segment-level feature rep...
specific Embodiment approach 2
[0022] Embodiment 2: This embodiment is different from Embodiment 1 in that Step 1 specifically includes the following steps:
[0023] Step 11. Extract segment-level time-frequency features from the training set and the audio to be represented:
[0024] Extract its 120-dimensional logarithmic Mel energy spectrum (Log-Mel) for each audio sample; then cut the logarithmic Mel energy spectrum of each sample into multiple logarithmic Mel energy spectrum fragments of equal length, namely , segment-level time-frequency features; there is 50% overlap between adjacent segments; obtain the segment-level time-frequency feature set of the training set and the segment-level time-frequency feature set of the audio to be represented;
[0025] Step 12, CNN network training:
[0026] Normalize the segments in the segment-level time-frequency feature set of the training set obtained in step 1. The specific method is to calculate the mean and standard deviation of the segments in the set, and t...
specific Embodiment approach 3
[0030] Specific embodiment three: the difference between this embodiment and specific embodiment two is that the CNN network includes two convolutional layers (Conv), three pooling layers (Pool), three fully connected layers (FC) and an output Layer, wherein, each convolutional layer and fully connected layer have all carried out the batch normalization operation, and the last (the third) pooling layer is full-time domain (complete time domain) pooling; Described CNN network adopts can The leaky linear rectification activation function Leaky ReLU is used to retain the negative part of the output vector of each layer, so as to ensure that the CNN network can retain as much dynamic information as possible. The specific network architecture and training parameter settings are shown in Table 1:
[0031] Table 1. CNN network architecture and training parameter settings
[0032]
[0033]As shown in Table 1, each Conv layer (convolutional layer) and FC layer (full connection laye...
PUM
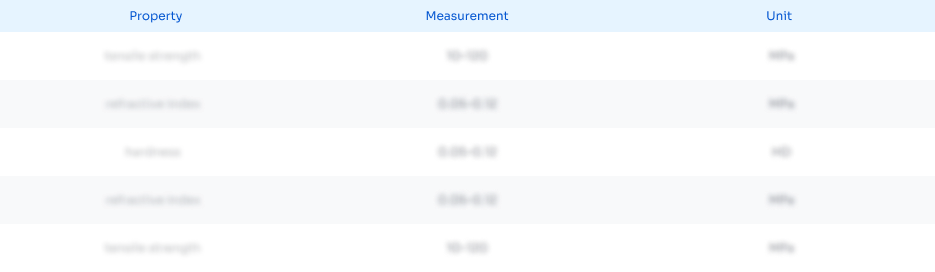
Abstract
Description
Claims
Application Information
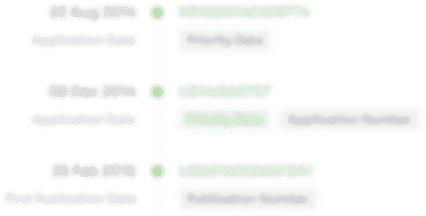
- R&D Engineer
- R&D Manager
- IP Professional
- Industry Leading Data Capabilities
- Powerful AI technology
- Patent DNA Extraction
Browse by: Latest US Patents, China's latest patents, Technical Efficacy Thesaurus, Application Domain, Technology Topic.
© 2024 PatSnap. All rights reserved.Legal|Privacy policy|Modern Slavery Act Transparency Statement|Sitemap