Semantic similarity calculation method based on deep learning
A semantic similarity and deep learning technology, applied in the field of semantic similarity calculation, can solve problems such as imperfect model feature extraction, low accuracy of similarity calculation, shallow network layers, etc., to overcome the problem of gradient disappearance and feature semantic information The effect of enriching and enhancing feature extraction capabilities
- Summary
- Abstract
- Description
- Claims
- Application Information
AI Technical Summary
Problems solved by technology
Method used
Image
Examples
Embodiment 1
[0048] Such as Figure 1-5 As shown, the present invention includes four steps: training data set construction, network model construction, model training and model prediction. The construction of the training data set and the construction of the network model are used as the basis for model training. After the model is trained, the trained model is used to calculate the semantic similarity.
[0049] 1.1 Manually construct the training data set. Each piece of data in the data set maintains a uniform format. In this application, the format is "text 1 text 2 label", and each piece of data consists of two texts, namely "text 1" and "text 2" and It consists of a label, the data example is as follows: "I want to modify the bound mobile phone number, how should I modify the bound mobile phone number 1", and separate "Text 1", "Text 2" and "Label" in each piece of data. Table symbol, if the label is 1, the two texts are similar texts, and if the label is 0, the piece of data is non-si...
PUM
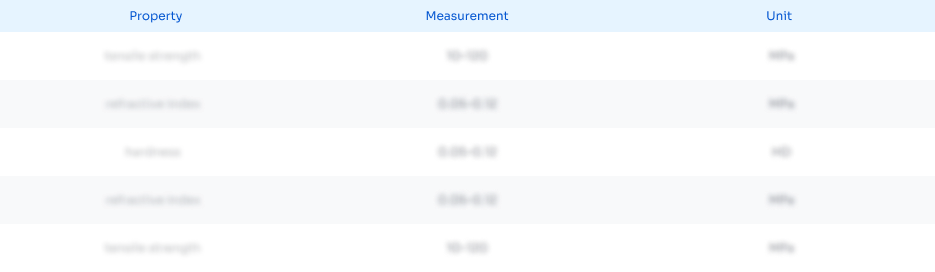
Abstract
Description
Claims
Application Information
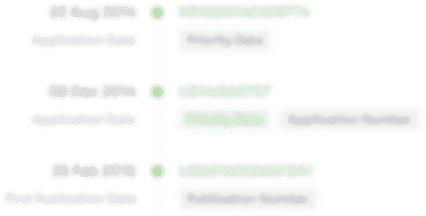
- R&D Engineer
- R&D Manager
- IP Professional
- Industry Leading Data Capabilities
- Powerful AI technology
- Patent DNA Extraction
Browse by: Latest US Patents, China's latest patents, Technical Efficacy Thesaurus, Application Domain, Technology Topic, Popular Technical Reports.
© 2024 PatSnap. All rights reserved.Legal|Privacy policy|Modern Slavery Act Transparency Statement|Sitemap|About US| Contact US: help@patsnap.com