Weakly-Supervised Image Semantic Segmentation Method Based on Candidate Regions and Neighborhood Classifiers
A candidate region and semantic segmentation technology, applied in the field of computer vision, can solve the problem of insufficient semantic label inference, and achieve the effect of ensuring accuracy, improving accuracy and improving accuracy.
- Summary
- Abstract
- Description
- Claims
- Application Information
AI Technical Summary
Problems solved by technology
Method used
Image
Examples
Embodiment Construction
[0037] figure 1 The overall processing flow of the present invention is given, which is combined below figure 1 The present invention is further described.
[0038] The present invention provides a weakly supervised image semantic segmentation method based on high-precision candidate regions and neighborhood classifiers. The main steps are described as follows:
[0039] Step 1. First, perform linear spectral clustering superpixel segmentation on the training image to obtain the desired superpixels, and then combine superpixels based on visual features until the number of combined superpixels is equal to the multiple of the number of labels contained in the image-level label, and obtain A collection of training images consisting of candidate regions.
[0040] Compared with superpixels as the basic processing unit, the number of candidate regions in the image is smaller, which is more helpful for improving the accuracy of semantic label inference. In addition, the candidate r...
PUM
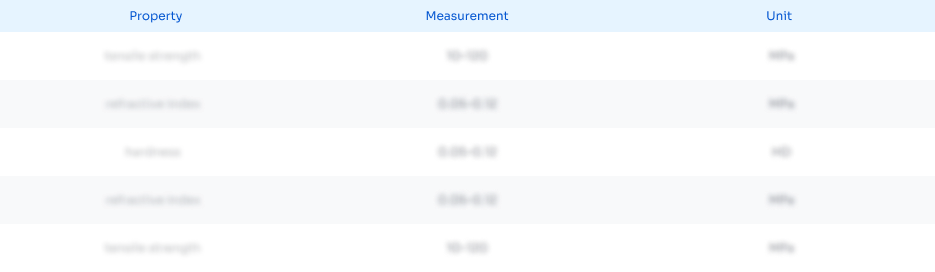
Abstract
Description
Claims
Application Information
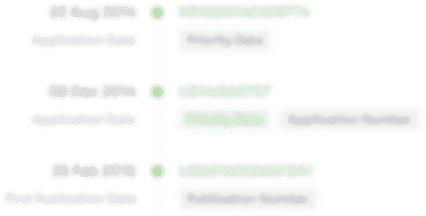
- R&D Engineer
- R&D Manager
- IP Professional
- Industry Leading Data Capabilities
- Powerful AI technology
- Patent DNA Extraction
Browse by: Latest US Patents, China's latest patents, Technical Efficacy Thesaurus, Application Domain, Technology Topic, Popular Technical Reports.
© 2024 PatSnap. All rights reserved.Legal|Privacy policy|Modern Slavery Act Transparency Statement|Sitemap|About US| Contact US: help@patsnap.com