Laser radar point cloud multi-target ground object identification method based on deep learning
A ground object recognition and lidar technology, applied in the field of point cloud recognition, can solve the problems of difficulty in feature extraction, large amount of calculation, low recognition accuracy, etc., and achieve the effect of reducing the amount of calculation, reducing the input dimension, and reducing the amount of calculation.
- Summary
- Abstract
- Description
- Claims
- Application Information
AI Technical Summary
Problems solved by technology
Method used
Image
Examples
Embodiment 1
[0044] A deep learning-based laser radar point cloud multi-target object recognition method includes the following steps:
[0045] a: Establish a data set: After the point cloud scene is segmented into regions, feature representations, and labels in sequence, point cloud data including several three-dimensional spaces are obtained. The point cloud data includes training sets and test sets, such as figure 2 Shown:
[0046] a1: Region segmentation: First calculate the minimum value of the XYZ coordinates in the point cloud scene, and then make a difference between each coordinate point and the minimum value of each coordinate axis to complete the translation of all coordinate points, and then each coordinate axis The area is divided into a unit of 100 meters, and divided into multiple small areas with a size of 100*100*100. For each small area, a number of three-dimensional spaces with a size of A*A*A are further subdivided with the parameter space size A; in this way, The ent...
Embodiment 2
[0071] A deep learning-based laser radar point cloud multi-target object recognition method includes the following steps:
[0072] Create a dataset:
[0073] Region segmentation: Find the minimum and maximum values of the x coordinates, the minimum and maximum values of the y coordinates and the minimum and maximum values of the z coordinates in the point cloud data, and subtract all the points from the minimum values of the corresponding coordinates to make the point cloud The data is in the range of 0-(max-min), which is convenient for subsequent data processing and improves the efficiency of program operation. Divide all point cloud data from top to bottom and from left to right into cubes of 100m*100m*100, the total number of regions is M*N*K (where M=int(max.x) / 100+1; N =int(max.y) / 100+1; K=int(max.z) / 100+1), traverse all the point cloud data in sequence and divide by 100 to round into the corresponding area, and traverse all Area, save the area where the point ...
PUM
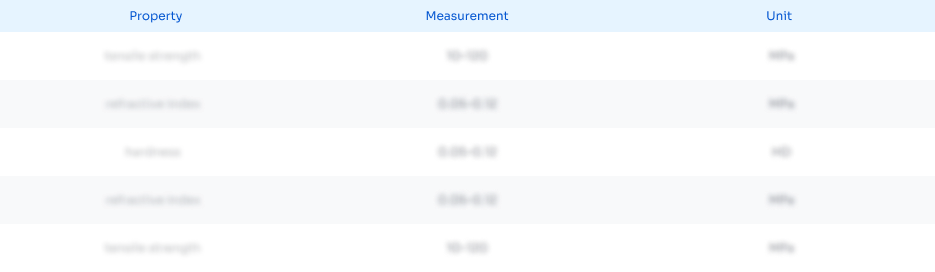
Abstract
Description
Claims
Application Information
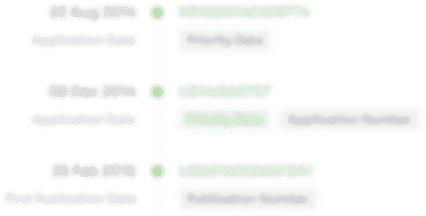
- R&D Engineer
- R&D Manager
- IP Professional
- Industry Leading Data Capabilities
- Powerful AI technology
- Patent DNA Extraction
Browse by: Latest US Patents, China's latest patents, Technical Efficacy Thesaurus, Application Domain, Technology Topic, Popular Technical Reports.
© 2024 PatSnap. All rights reserved.Legal|Privacy policy|Modern Slavery Act Transparency Statement|Sitemap|About US| Contact US: help@patsnap.com