Deep residual steganalysis method based on heterogeneous kernel
A steganalysis and heterogeneous core technology, applied in the field of content security, can solve the problems of limited image size, long training time, poor versatility, etc., to reduce interference, ensure accuracy, and improve versatility.
- Summary
- Abstract
- Description
- Claims
- Application Information
AI Technical Summary
Problems solved by technology
Method used
Image
Examples
Example Embodiment
[0034] The present invention will be further described below through the drawings and embodiments.
[0035] A deep residual steganalysis method based on heterogeneous cores. The SRM model is used in the preprocessing stage of ResNets, and the HetConv heterogeneous core is used as a convolution core in the DRHNet network. It includes the following steps:
[0036] Step 1. Extract the feature vector of each SRM sub-model of the image;
[0037] Step 2. Combine and reorganize all the feature vectors of the image;
[0038] Step 3. Put the original image and the encrypted image into the DRHNet network model for training;
[0039] Step 4. Put the image to be detected into the trained DRHNet steganalysis network for detection, and the result is used as a reference for whether the image is embedded.
[0040] Specifically, step 1. The SRM model uses k high-pass filters to extract the residuals of the overlay image or the hidden image to form k sub-models; then each sub-model is quantized, rounded, ...
PUM
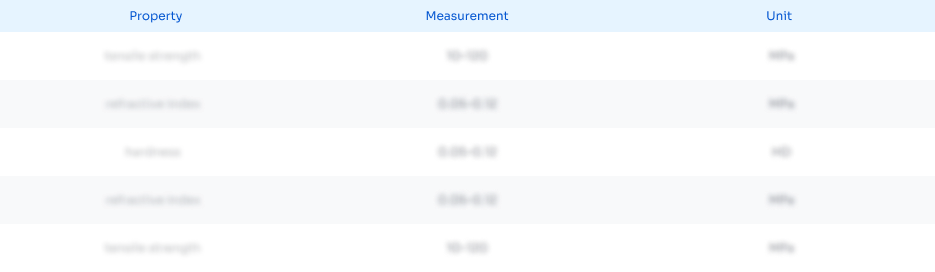
Abstract
Description
Claims
Application Information
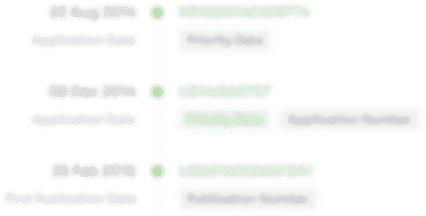
- R&D Engineer
- R&D Manager
- IP Professional
- Industry Leading Data Capabilities
- Powerful AI technology
- Patent DNA Extraction
Browse by: Latest US Patents, China's latest patents, Technical Efficacy Thesaurus, Application Domain, Technology Topic, Popular Technical Reports.
© 2024 PatSnap. All rights reserved.Legal|Privacy policy|Modern Slavery Act Transparency Statement|Sitemap|About US| Contact US: help@patsnap.com