Deep neural network hyper-parameter optimization method, electronic device and storage medium
A technology of deep neural network and optimization method, applied in the direction of neural learning method, biological neural network model, neural architecture, etc., can solve the problems of long running time and high computing cost, and achieve the effect of reducing computing cost and running time cost
- Summary
- Abstract
- Description
- Claims
- Application Information
AI Technical Summary
Problems solved by technology
Method used
Image
Examples
Embodiment 1
[0038] The present invention proposes a conditional neural network process (Conditional Neural Processes, CNPs) to replace the Gaussian Process (Gaussian Process, GPs), and the CNPs combines the characteristics of the random process and the neural network, inspired by the flexibility of the Gaussian process and using Gradient descent is used to train the neural network, and then realize the hyperparameter optimization method.
[0039] The conditional neural network process is parameterized by the neural network when learning from known observation data. The conditional neural network model is trained by randomly sampling the data set and following the gradient step to maximize the random sub- The conditional likelihood of the set.
[0040] The general method of hyperparameter optimization in the prior art is to use Gaussian process for model training, but this patent uses a conditional neural network model with stronger learning ability to replace the traditional Gaussian proc...
PUM
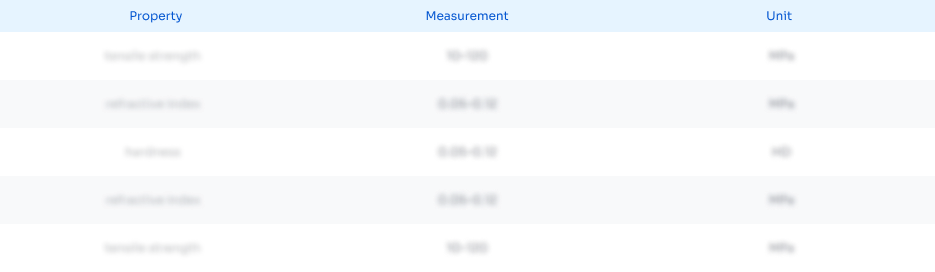
Abstract
Description
Claims
Application Information
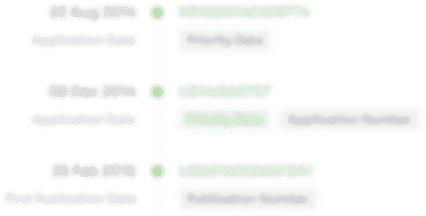
- R&D
- Intellectual Property
- Life Sciences
- Materials
- Tech Scout
- Unparalleled Data Quality
- Higher Quality Content
- 60% Fewer Hallucinations
Browse by: Latest US Patents, China's latest patents, Technical Efficacy Thesaurus, Application Domain, Technology Topic, Popular Technical Reports.
© 2025 PatSnap. All rights reserved.Legal|Privacy policy|Modern Slavery Act Transparency Statement|Sitemap|About US| Contact US: help@patsnap.com