Multiple vehicle trajectory prediction method based on long-short memory network
A vehicle trajectory and prediction method technology, applied in prediction, neural learning methods, biological neural network models, etc., can solve problems such as low efficiency, inconformity with sensor perspectives, and results that cannot be applied to actual situations, and achieve the effect of improving prediction accuracy
- Summary
- Abstract
- Description
- Claims
- Application Information
AI Technical Summary
Problems solved by technology
Method used
Image
Examples
Embodiment
[0070] In this embodiment, the specific hyperparameters of the neural network are shown in the following table:
[0071]
[0072] Such as figure 2 As shown in the figure, the unmanned vehicle is the main vehicle (vehicle labeled (0)) and 6 surrounding vehicles (6 vehicles labeled (1)-(6)), and the origin O of the coordinate system is at the main vehicle. The geometric center of the car, the y-axis is along the tangent direction of the road's forward direction, and the x-axis is perpendicular to the y-axis, pointing from the left edge line of the road to the right edge line.
[0073] Such as image 3 As shown, in the three scenarios based on the method of long short memory network encoder decoder structure ( Figure 3-1 , Figure 3-2 , Figure 3-3 ) The trajectory prediction true value and predicted value results of the vehicle. Among them, each test scenario contains the test results of vehicles labeled (1)-(6). In order to show the generalization ability of the netw...
PUM
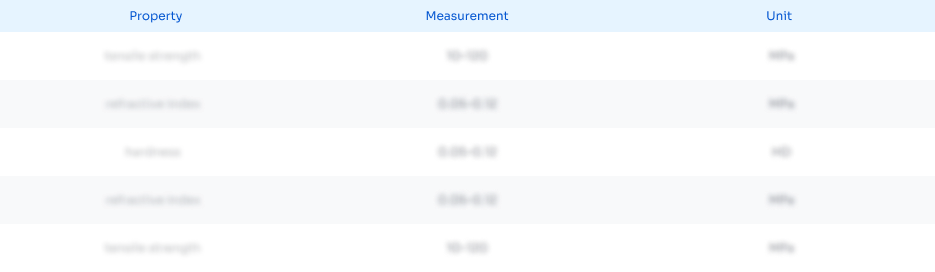
Abstract
Description
Claims
Application Information
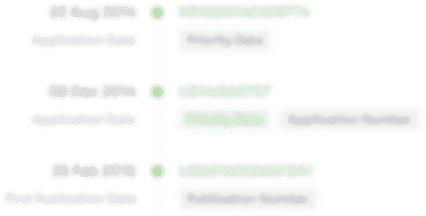
- R&D
- Intellectual Property
- Life Sciences
- Materials
- Tech Scout
- Unparalleled Data Quality
- Higher Quality Content
- 60% Fewer Hallucinations
Browse by: Latest US Patents, China's latest patents, Technical Efficacy Thesaurus, Application Domain, Technology Topic, Popular Technical Reports.
© 2025 PatSnap. All rights reserved.Legal|Privacy policy|Modern Slavery Act Transparency Statement|Sitemap|About US| Contact US: help@patsnap.com