Method for representing and generalization of robot discrete track based on probability model
A probabilistic model, discrete technology, applied in manipulators, program-controlled manipulators, manufacturing tools, etc., can solve problems such as a lot of time costs, cumbersome teaching strategies, lack of trajectory splicing strategies, etc., to achieve a simple and operable teaching process. strong effect
- Summary
- Abstract
- Description
- Claims
- Application Information
AI Technical Summary
Problems solved by technology
Method used
Image
Examples
Embodiment Construction
[0035] Below in conjunction with accompanying drawing and embodiment example, the present invention will be further described:
[0036] As shown in the figure: a probabilistic model-based representation and generalization method for discrete robot trajectories, including:
[0037] (1) Teaching of discrete trajectory:
[0038] The source of the teaching data is obtained by dragging the teaching strategy, and the teaching data is represented first: for the two-dimensional teaching data, this paper expresses it as:
[0039]
[0040] Among them, y i,s ,y i,t respectively represent the spatial information and time information of the teaching track, and T represents the number of teaching points in the teaching track.
[0041] (2), for discrete trajectory representation and parameter learning:
[0042] For the multidimensional teaching variable y, the modeling GMM is:
[0043]
[0044] Among them, p(y) represents the probability density function, N(y, μ k , ∑ k ) expres...
PUM
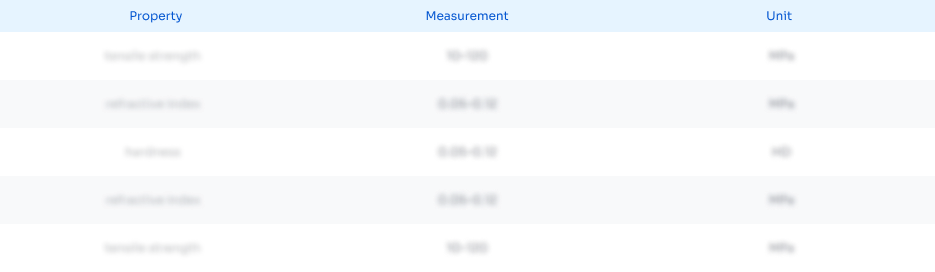
Abstract
Description
Claims
Application Information
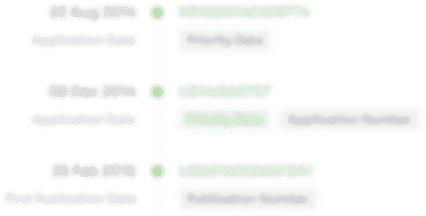
- R&D
- Intellectual Property
- Life Sciences
- Materials
- Tech Scout
- Unparalleled Data Quality
- Higher Quality Content
- 60% Fewer Hallucinations
Browse by: Latest US Patents, China's latest patents, Technical Efficacy Thesaurus, Application Domain, Technology Topic, Popular Technical Reports.
© 2025 PatSnap. All rights reserved.Legal|Privacy policy|Modern Slavery Act Transparency Statement|Sitemap|About US| Contact US: help@patsnap.com