Cluster photovoltaic power probability prediction method and system, medium and electronic equipment
A probabilistic forecasting and photovoltaic technology, applied in forecasting, data processing applications, instruments, etc., can solve the problem of inability to provide more accurate regional photovoltaic power probability forecasting information, the reduction of cluster photovoltaic power generation forecast accuracy, and research on cluster photovoltaic power probability forecasting Fewer problems, such as shortening training time, accurate feature extraction, and reducing computing costs
- Summary
- Abstract
- Description
- Claims
- Application Information
AI Technical Summary
Problems solved by technology
Method used
Image
Examples
Embodiment 1
[0058] For the probabilistic prediction of cluster photovoltaic power, the prediction method needs to process the input data of all photovoltaic stations in the area (such as the numerical weather forecast information and historical power generation of each photovoltaic station, etc.), how to effectively extract features from a large amount of input data Probabilistic forecasting methods for cluster photovoltaic power are crucial.
[0059] Convolutional Neural Network (CNN) is an effective feature extraction technique for deep learning. The convolutional neural network-quantile regression model (CNN-QR) has been applied in the probability prediction of a single photovoltaic station, and the prediction effect is very good. As the core of the model, the convolutional neural network is used to learn the nonlinear quantile regression function, which establishes the nonlinear mapping relationship between the input information and the output quantile of a single photovoltaic site. ...
Embodiment 2
[0135] Embodiment 2 of the present disclosure provides a system for probabilistic prediction of cluster photovoltaic power, including:
[0136] The data acquisition module is configured to: collect historical data of each photovoltaic field station, and perform normalization processing on the collected historical data;
[0137] The data processing module is configured to: use the improved convolutional neural network-quantile regression model to extract representative features from the input data of a single photovoltaic station, and then comprehensively extract the correlation between photovoltaic stations in the area feature;
[0138] The prediction module is configured to: the improved convolutional neural network-quantile regression model outputs a quantile prediction result of regional photovoltaic power generation according to the extracted correlation characteristics between photovoltaic stations in the region.
[0139] The historical data of each photovoltaic station ...
Embodiment 3
[0152] Embodiment 3 of the present disclosure provides a readable storage medium on which a program is stored, and when the program is executed by a processor, the steps in the probabilistic prediction of cluster photovoltaic power described in Embodiment 1 of the present disclosure are implemented.
PUM
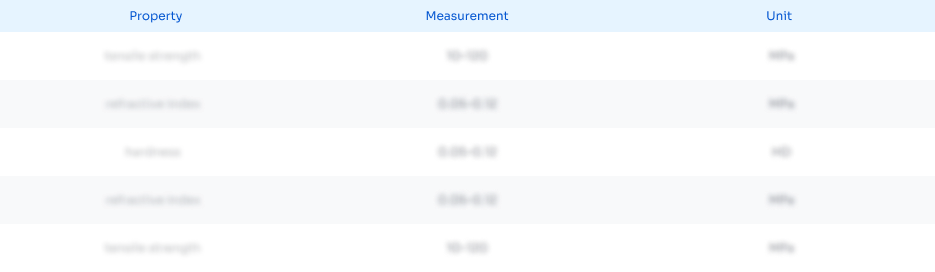
Abstract
Description
Claims
Application Information
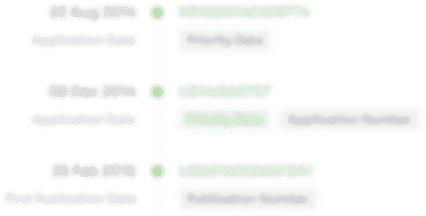
- R&D
- Intellectual Property
- Life Sciences
- Materials
- Tech Scout
- Unparalleled Data Quality
- Higher Quality Content
- 60% Fewer Hallucinations
Browse by: Latest US Patents, China's latest patents, Technical Efficacy Thesaurus, Application Domain, Technology Topic, Popular Technical Reports.
© 2025 PatSnap. All rights reserved.Legal|Privacy policy|Modern Slavery Act Transparency Statement|Sitemap|About US| Contact US: help@patsnap.com