Electroencephalogram signal classification method based on graph semi-supervised width learning
A technology of EEG signals and classification methods, applied in the field of pattern recognition, can solve problems such as complex structure adjustment and massive calculations
- Summary
- Abstract
- Description
- Claims
- Application Information
AI Technical Summary
Problems solved by technology
Method used
Image
Examples
Embodiment Construction
[0049] The present invention is described in detail below in conjunction with accompanying drawing based on graph semi-supervised width learning classification method, figure 1 for the implementation flow chart.
[0050] Such as figure 1 , the implementation of the method of the present invention mainly includes four steps: (1) extending the labels of labeled samples to unlabeled samples through graphs to obtain corresponding pseudo-labels; (2) optimizing and solving the objective function of the graph constructed, and further Improve the accuracy of pseudo-labels; (3) send labeled samples and unlabeled samples and corresponding labels and pseudo-labels to BLS to build SS-BLS models; (4) test the constructed semi-supervised model and compare with Compare with other algorithms.
[0051] Each step will be described in detail below one by one.
[0052] Step (1), based on the premise of graph label extension, assumes that the closer the samples are in the feature space, the mor...
PUM
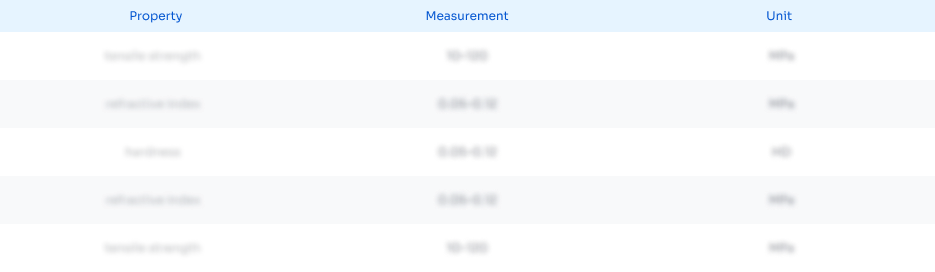
Abstract
Description
Claims
Application Information
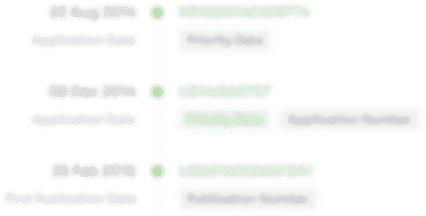
- R&D Engineer
- R&D Manager
- IP Professional
- Industry Leading Data Capabilities
- Powerful AI technology
- Patent DNA Extraction
Browse by: Latest US Patents, China's latest patents, Technical Efficacy Thesaurus, Application Domain, Technology Topic, Popular Technical Reports.
© 2024 PatSnap. All rights reserved.Legal|Privacy policy|Modern Slavery Act Transparency Statement|Sitemap|About US| Contact US: help@patsnap.com