Unsupervised transfer learning method based on graph convolution network
A convolutional network and migration learning technology, which is applied to instruments, character and pattern recognition, computer components, etc., can solve the problems of data distribution differences between the source domain and the target domain, ignore data geometric information, unsupervised migration learning, etc., to achieve Strong discrimination, improved accuracy, and good migration performance
- Summary
- Abstract
- Description
- Claims
- Application Information
AI Technical Summary
Problems solved by technology
Method used
Image
Examples
Embodiment
[0041] Such as figure 1 , figure 2 As shown, an unsupervised transfer learning method based on graph convolutional network includes the following steps:
[0042] S1: Obtain the source domain and target domain samples for transfer learning from the database, perform feature extraction on the data samples, that is, source domain samples and target domain samples, and construct a correlation diagram between each data sample based on the nearest neighbor algorithm model; The source domain and target domain samples in this embodiment are computer monitor pictures from different places respectively, wherein, the source domain pictures are from online shopping malls, and the target domain pictures are from ordinary cameras, and their distributions are obviously different; The trained AlexNet network model extracts the features of these pictures, and uses the k-nearest neighbor algorithm to construct a correlation diagram between each data sample;
[0043] S2: Put the sample featur...
PUM
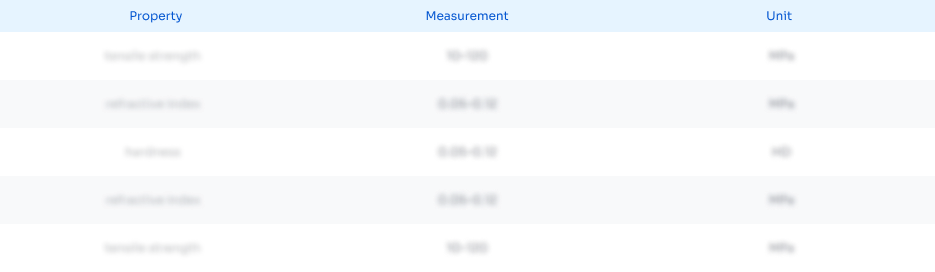
Abstract
Description
Claims
Application Information
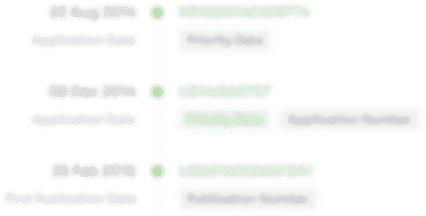
- R&D Engineer
- R&D Manager
- IP Professional
- Industry Leading Data Capabilities
- Powerful AI technology
- Patent DNA Extraction
Browse by: Latest US Patents, China's latest patents, Technical Efficacy Thesaurus, Application Domain, Technology Topic.
© 2024 PatSnap. All rights reserved.Legal|Privacy policy|Modern Slavery Act Transparency Statement|Sitemap