Hyperspectral image spectral space classification method based on class characteristic iterative random sampling
A hyperspectral image and iterative technology, applied to computer parts, instruments, character and pattern recognition, etc., can solve the problems of poor classification effect, high cost and laboriousness of ICEM
- Summary
- Abstract
- Description
- Claims
- Application Information
AI Technical Summary
Problems solved by technology
Method used
Image
Examples
Embodiment
[0104] The specific steps of the hyperspectral image spectral space classification method based on class feature iterative random sampling implemented by the present invention are as follows:
[0105] A. Sample data source: The sample hyperspectral data comes from the hyperspectral data of the IndianPine experimental area in Indian State, USA provided by Purdue University. This data has 220 bands, and its 186th band image is as follows figure 2 shown. The size of the image data is 145×145 pixels, including 16 types of ground objects, a total of 10249 pixel points, and its distribution and categories are as follows image 3 shown.
[0106] B. Class feature extraction: According to the formulas (1) and (2), respectively calculate the class feature probabilities of the 16 types of ground objects in the above-mentioned Indian Pine experimental area, specifically the class feature probabilities p of the 16 types of ground objects CD and value p SR As shown in Table 1.
[0107]...
PUM
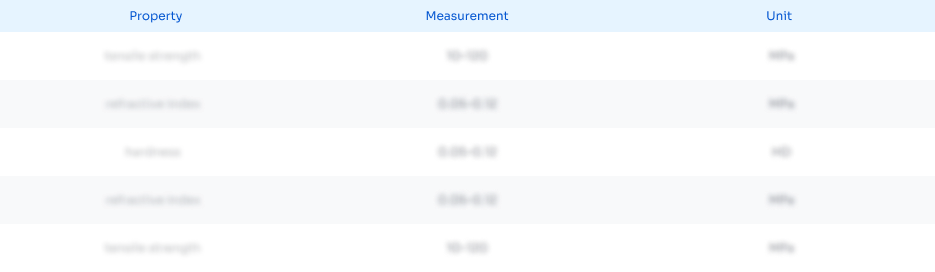
Abstract
Description
Claims
Application Information
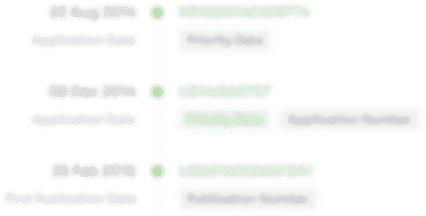
- R&D Engineer
- R&D Manager
- IP Professional
- Industry Leading Data Capabilities
- Powerful AI technology
- Patent DNA Extraction
Browse by: Latest US Patents, China's latest patents, Technical Efficacy Thesaurus, Application Domain, Technology Topic, Popular Technical Reports.
© 2024 PatSnap. All rights reserved.Legal|Privacy policy|Modern Slavery Act Transparency Statement|Sitemap|About US| Contact US: help@patsnap.com