A method for predicting vehicle queuing dissipation time based on image self-learning
A technology of dissipation time and prediction method, which is applied in the field of intelligent transportation, can solve the problems of not being able to obtain all signals at intersections, limited sensing range, and damaged coils, and achieve the effects of improving prediction accuracy, improving prediction accuracy, and ensuring reliability
- Summary
- Abstract
- Description
- Claims
- Application Information
AI Technical Summary
Problems solved by technology
Method used
Image
Examples
Embodiment Construction
[0069] The present invention will be described in detail below in conjunction with the accompanying drawings and specific embodiments.
[0070] Such as figure 1 As shown, a method for predicting vehicle queuing dissipation time based on image self-learning includes the following steps:
[0071] S1. Pre-set the analysis area of each lane of the entrance road;
[0072] S2. Based on the analysis area of each lane of the entrance road, at the preset photographing time, the image of the entrance road is collected through the video acquisition equipment arranged at the entrance road;
[0073] S3. Carry out image segmentation on the entrance road image, divide each lane from the entrance road image, and obtain the lane image corresponding to each lane;
[0074] S4. Establish a self-learning neural network, analyze the image of the lane by the self-learning neural network, and obtain the vehicle queuing and dissipation prediction time of the lane;
[0075] S5. Acquiring the act...
PUM
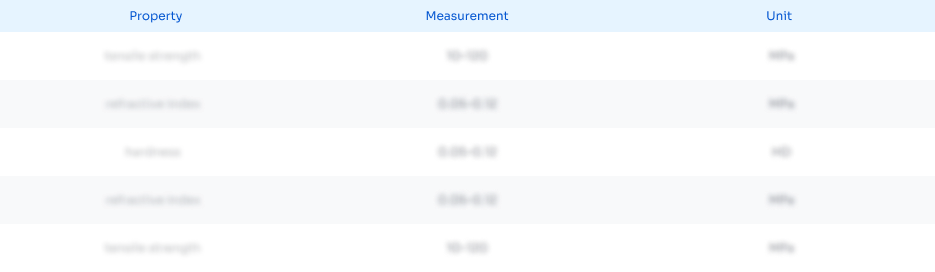
Abstract
Description
Claims
Application Information
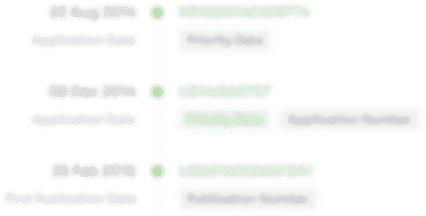
- R&D Engineer
- R&D Manager
- IP Professional
- Industry Leading Data Capabilities
- Powerful AI technology
- Patent DNA Extraction
Browse by: Latest US Patents, China's latest patents, Technical Efficacy Thesaurus, Application Domain, Technology Topic.
© 2024 PatSnap. All rights reserved.Legal|Privacy policy|Modern Slavery Act Transparency Statement|Sitemap