N-gamma discrimination method based on semi-supervised support vector machine
A support vector machine and semi-supervised technology, applied in computer parts, character and pattern recognition, measurement with scintillation detectors, etc., can solve problems such as huge network, inability to obtain standard samples, complex structure, etc., and reduce training samples Requirements for scale, reducing time and computing power overhead, and ensuring consistency
- Summary
- Abstract
- Description
- Claims
- Application Information
AI Technical Summary
Problems solved by technology
Method used
Image
Examples
Embodiment 1
[0051] A kind of n-γ discrimination method based on semi-supervised support vector machine, it is characterized in that comprising the following steps:
[0052] a) Use an analog-to-digital converter to perform analog-to-digital conversion on the output pulse signal of the detector, collect the digitized detector pulse signal, and form a training data set; use the training data set combined with a semi-supervised learning method to train a support vector machine, and obtain a Optimal classification hyperplane;
[0053] b) Digitize the newly detected pulse and perform feature extraction preprocessing: extract important feature information of the pulse signal;
[0054] c) Input the extracted feature information into the support vector machine, and use the above-mentioned classification hyperplane combined with the above-mentioned extracted features to classify and predict the newly detected pulse samples.
[0055] The detailed step of said step a) is to use an analog-to-digital ...
Embodiment 2
[0062] A kind of n-γ discrimination method based on semi-supervised support vector machine, it is characterized in that comprising the following steps:
[0063] a) Use an analog-to-digital converter to perform analog-to-digital conversion on the output pulse signal of the detector, collect the digitized detector pulse signal, and form a training data set; use the training data set combined with a semi-supervised learning method to train a support vector machine, and obtain a Optimal classification hyperplane;
[0064] b) Digitize the newly detected pulse and perform feature extraction preprocessing: extract important feature information of the pulse signal;
[0065] c) Input the extracted feature information into the support vector machine, and use the above-mentioned classification hyperplane combined with the above-mentioned extracted features to classify and predict the newly detected pulse samples.
[0066] The detailed step of said step a) is to use an analog-to-digital ...
Embodiment 3
[0069] A kind of n-γ discrimination method based on semi-supervised support vector machine, it is characterized in that comprising the following steps:
[0070] a) Use an analog-to-digital converter to perform analog-to-digital conversion on the output pulse signal of the detector, collect the digitized detector pulse signal, and form a training data set; use the training data set combined with a semi-supervised learning method to train a support vector machine, and obtain a Optimal classification hyperplane;
[0071] b) Digitize the newly detected pulse and perform feature extraction preprocessing: extract important feature information of the pulse signal;
[0072] c) Input the extracted feature information into the support vector machine, and use the above-mentioned classification hyperplane combined with the above-mentioned extracted features to classify and predict the newly detected pulse samples.
[0073] The detailed step of said step a) is to use an analog-to-digital ...
PUM
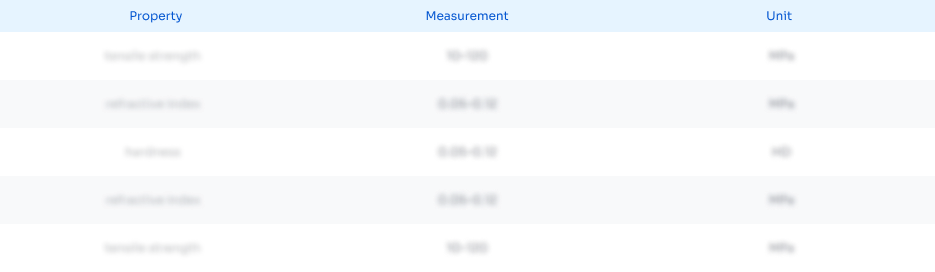
Abstract
Description
Claims
Application Information
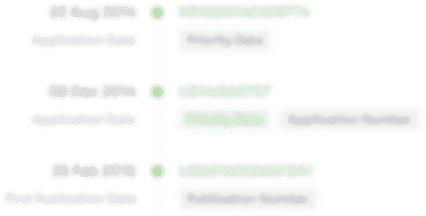
- R&D
- Intellectual Property
- Life Sciences
- Materials
- Tech Scout
- Unparalleled Data Quality
- Higher Quality Content
- 60% Fewer Hallucinations
Browse by: Latest US Patents, China's latest patents, Technical Efficacy Thesaurus, Application Domain, Technology Topic, Popular Technical Reports.
© 2025 PatSnap. All rights reserved.Legal|Privacy policy|Modern Slavery Act Transparency Statement|Sitemap|About US| Contact US: help@patsnap.com