Human body behavior recognition method and system based on graph convolution network
A technology of convolutional network and recognition method, applied in neural learning method, character and pattern recognition, biological neural network model, etc., can solve the problems of lack of modeling of long-term time relationship of topological sequence and insufficient learning ability
- Summary
- Abstract
- Description
- Claims
- Application Information
AI Technical Summary
Problems solved by technology
Method used
Image
Examples
Embodiment Construction
[0056] The technical solutions in the embodiments of the present invention will be clearly and completely described below in conjunction with the embodiments of the present invention. Apparently, the described embodiments are only some of the embodiments of the present invention, not all of them. Based on the embodiments of the present invention, all other embodiments obtained by persons of ordinary skill in the art without making creative efforts belong to the protection scope of the present invention.
[0057] Embodiments of the present invention provide a human behavior recognition method based on spatio-temporal graph convolution and graph convolution long-short-term memory network, such as figure 1 shown, including the following steps:
[0058] S1, extract the human skeleton information from the image containing human behavior, obtain the human body joint point position information sequence, use each joint point as a node, and use the bones between the joint points as edg...
PUM
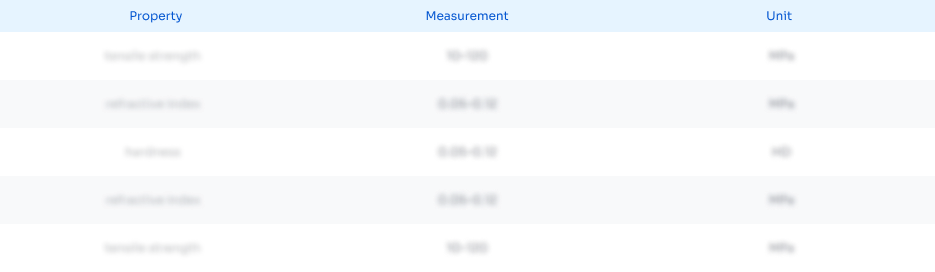
Abstract
Description
Claims
Application Information
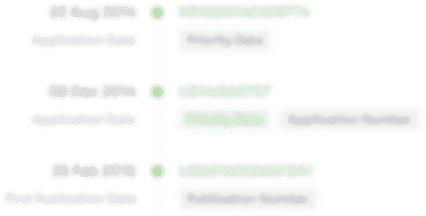
- R&D
- Intellectual Property
- Life Sciences
- Materials
- Tech Scout
- Unparalleled Data Quality
- Higher Quality Content
- 60% Fewer Hallucinations
Browse by: Latest US Patents, China's latest patents, Technical Efficacy Thesaurus, Application Domain, Technology Topic, Popular Technical Reports.
© 2025 PatSnap. All rights reserved.Legal|Privacy policy|Modern Slavery Act Transparency Statement|Sitemap|About US| Contact US: help@patsnap.com