Time sequence classification method based on deep reinforcement learning
A technology of time series and reinforcement learning, applied in neural learning methods, instruments, biological neural network models, etc., can solve problems such as high computational complexity and low accuracy of time series classification decision-making, achieve low computational complexity and improve convergence speed , high robustness effect
- Summary
- Abstract
- Description
- Claims
- Application Information
AI Technical Summary
Problems solved by technology
Method used
Image
Examples
Embodiment Construction
[0053] The specific embodiments of the present invention are described below so that those skilled in the art can understand the present invention, but it should be clear that the present invention is not limited to the scope of the specific embodiments. For those of ordinary skill in the art, as long as various changes Within the spirit and scope of the present invention defined and determined by the appended claims, these changes are obvious, and all inventions and creations using the concept of the present invention are included in the protection list.
[0054] Embodiments of the present invention will be described in detail below in conjunction with the accompanying drawings.
[0055] Such as figure 1 As shown, a time series classification method based on deep reinforcement learning includes the following steps:
[0056] S1. Collect several time series, obtain sample data, and preprocess the sample data;
[0057] S2. Construct a deep residual network, and update the deep r...
PUM
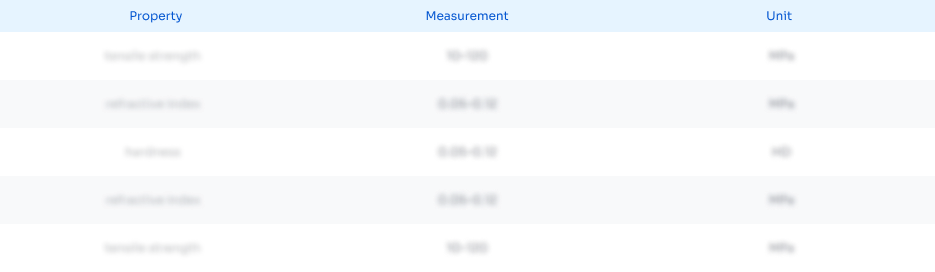
Abstract
Description
Claims
Application Information
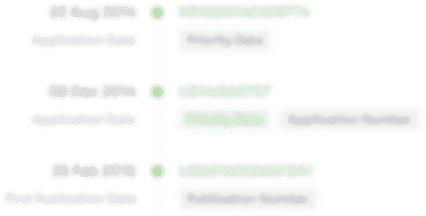
- R&D Engineer
- R&D Manager
- IP Professional
- Industry Leading Data Capabilities
- Powerful AI technology
- Patent DNA Extraction
Browse by: Latest US Patents, China's latest patents, Technical Efficacy Thesaurus, Application Domain, Technology Topic, Popular Technical Reports.
© 2024 PatSnap. All rights reserved.Legal|Privacy policy|Modern Slavery Act Transparency Statement|Sitemap|About US| Contact US: help@patsnap.com