Zero-sample image classification model based on repeated attention network and method thereof
A sample image and classification model technology, which is applied to computer components, character and pattern recognition, instruments, etc., can solve problems such as heavy workload, achieve effect improvement, image representation information is accurate, and the effect of alleviating the problem of strong bias
- Summary
- Abstract
- Description
- Claims
- Application Information
AI Technical Summary
Problems solved by technology
Method used
Image
Examples
Embodiment Construction
[0039] The present invention will be further described below with reference to the accompanying drawings and embodiments.
[0040] Please refer to figure 1 , the present invention provides a zero-sample image classification model based on repeated attention network, including
[0041] Repeated attention network module for training and obtaining image region sequence information;
[0042] Generative adversarial network module for obtaining visual error information;
[0043] The visual feature extraction network processing module is used to obtain the one-dimensional visual feature vector of the image;
[0044] The attribute semantic transformation network module uses two layers of linear activation layers to map the low-dimensional attribute semantic vector to the high-dimensional feature vector with the same dimension as the visual feature vector;
[0045] The visual-attribute semantic connection network realizes the fusion of visual feature vector and attribute semantic fe...
PUM
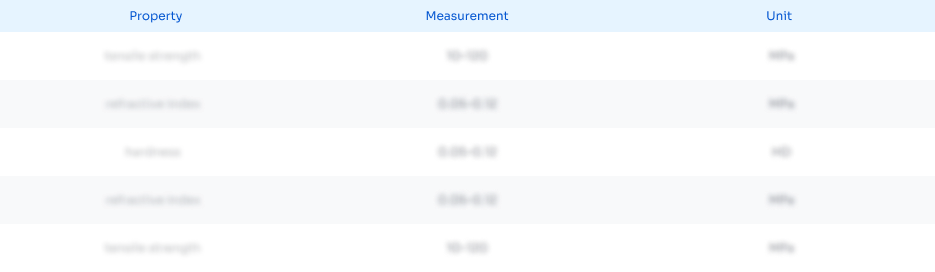
Abstract
Description
Claims
Application Information
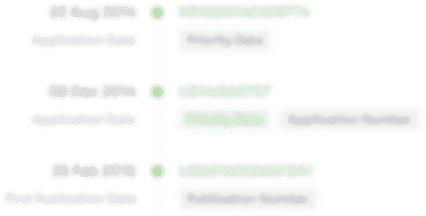
- R&D Engineer
- R&D Manager
- IP Professional
- Industry Leading Data Capabilities
- Powerful AI technology
- Patent DNA Extraction
Browse by: Latest US Patents, China's latest patents, Technical Efficacy Thesaurus, Application Domain, Technology Topic, Popular Technical Reports.
© 2024 PatSnap. All rights reserved.Legal|Privacy policy|Modern Slavery Act Transparency Statement|Sitemap|About US| Contact US: help@patsnap.com