Method for representing crowd movement patterns through context-dependent graph embedding
A technology of moving patterns and embedded representations, applied in neural learning methods, character and pattern recognition, biological neural network models, etc., can solve problems such as lack of labeled training data
- Summary
- Abstract
- Description
- Claims
- Application Information
AI Technical Summary
Problems solved by technology
Method used
Image
Examples
Embodiment
[0053] The method provided by this implementation to represent crowd movement patterns through context-sensitive graph embeddings can be used for such datasets containing user check-in records. The real-world LBSN dataset as shown in Table 1, with Gowalla ( http: / / snap.stanford.edu / data / loc-gowalla.html acquisition) as an example to experiment.
[0054] Table 1: Relevant information of experimental data of the present invention
[0055]
[0056] |u| indicates the number of users;
[0057] |D L | / |D U |respectively represent the number of sub-trajectories used for training and testing;
[0058] |L| represents the number of different check-in points in the dataset;
[0059] Represents the average length of the trajectory before it is divided;
[0060] T r Represents the range of trajectory lengths in the dataset;
[0061] New York represents the data that the area in the dataset Foursquare is New York;
[0062] Tokyo represents the data whose region is Tokyo in...
PUM
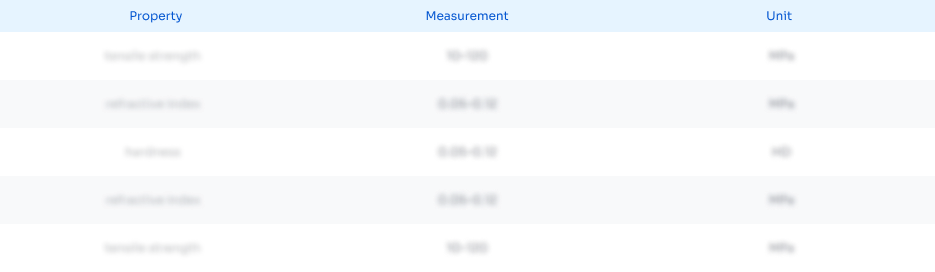
Abstract
Description
Claims
Application Information
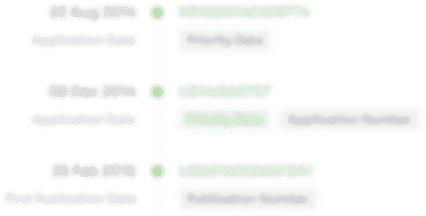
- R&D
- Intellectual Property
- Life Sciences
- Materials
- Tech Scout
- Unparalleled Data Quality
- Higher Quality Content
- 60% Fewer Hallucinations
Browse by: Latest US Patents, China's latest patents, Technical Efficacy Thesaurus, Application Domain, Technology Topic, Popular Technical Reports.
© 2025 PatSnap. All rights reserved.Legal|Privacy policy|Modern Slavery Act Transparency Statement|Sitemap|About US| Contact US: help@patsnap.com