Hyperspectral image classification method combining 3D/2D convolutional network and adaptive spectral unmixing
A hyperspectral image and spectral unmixing technology, applied in the field of hyperspectral image classification, can solve problems such as affecting the accuracy of hyperspectral classification, and achieve the effects of reducing complexity, enhancing feature learning, and high computational efficiency
- Summary
- Abstract
- Description
- Claims
- Application Information
AI Technical Summary
Problems solved by technology
Method used
Image
Examples
Embodiment
[0045] Step 1: Data preprocessing. The maximum and minimum normalization is performed on the hyperspectral image data to be processed, and the normalization formula is as follows:
[0046]
[0047] where x ijs Represents a pixel in the hyperspectral image, i and j respectively represent the coordinate position of the pixel in the hyperspectral image, s represents the spectral segment of the hyperspectral image, and the existing hyperspectral image generally contains 100-240 spectral segments , is a normalized pixel, x ··smax 、x ··smin Represent the maximum and minimum values of the 3D hyperspectral image in the s-band, respectively.
[0048] Step 2: Data Segmentation. Count the number of labeled samples in hyperspectral images, and divide the data into three parts: labeled training sample set X L , validation sample set X V , test sample X T . For a three-dimensional hyperspectral image data with a size of M*N*D, M and N represent the height and width of the hyp...
PUM
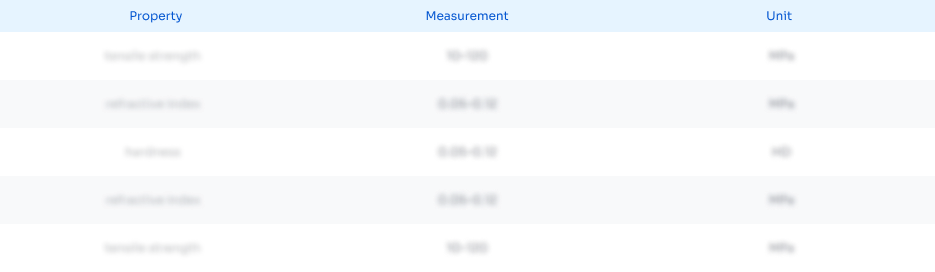
Abstract
Description
Claims
Application Information
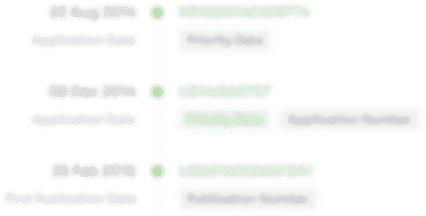
- R&D Engineer
- R&D Manager
- IP Professional
- Industry Leading Data Capabilities
- Powerful AI technology
- Patent DNA Extraction
Browse by: Latest US Patents, China's latest patents, Technical Efficacy Thesaurus, Application Domain, Technology Topic, Popular Technical Reports.
© 2024 PatSnap. All rights reserved.Legal|Privacy policy|Modern Slavery Act Transparency Statement|Sitemap|About US| Contact US: help@patsnap.com