Model generation method and device
A technology of model generation and quantification methods, applied in neural learning methods, biological neural network models, neural architectures, etc., can solve problems such as large loss of model accuracy, difficulty in achieving both model accuracy and operational efficiency, and loss of neural network model accuracy. Achieve the effect of improving search efficiency
- Summary
- Abstract
- Description
- Claims
- Application Information
AI Technical Summary
Problems solved by technology
Method used
Image
Examples
Embodiment Construction
[0023] The present disclosure will be further described in detail below in conjunction with the accompanying drawings and embodiments. It should be understood that the specific embodiments described here are only used to explain related inventions, rather than to limit the invention. It should also be noted that, for the convenience of description, only the parts related to the related invention are shown in the drawings.
[0024] It should be noted that, in the case of no conflict, the embodiments in the present disclosure and the features in the embodiments can be combined with each other. The present disclosure will be described in detail below with reference to the accompanying drawings and embodiments.
[0025] figure 1 An exemplary system architecture 100 to which the model generation method or model generation apparatus of the present disclosure can be applied is shown.
[0026] figure 1 An exemplary system architecture 100 to which the model generation method or mo...
PUM
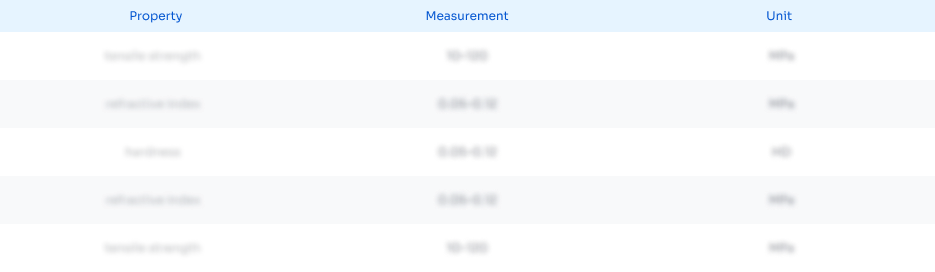
Abstract
Description
Claims
Application Information
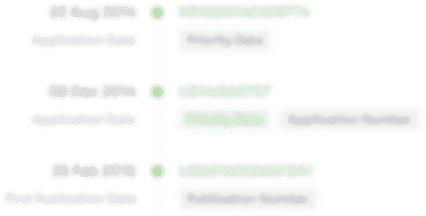
- R&D Engineer
- R&D Manager
- IP Professional
- Industry Leading Data Capabilities
- Powerful AI technology
- Patent DNA Extraction
Browse by: Latest US Patents, China's latest patents, Technical Efficacy Thesaurus, Application Domain, Technology Topic, Popular Technical Reports.
© 2024 PatSnap. All rights reserved.Legal|Privacy policy|Modern Slavery Act Transparency Statement|Sitemap|About US| Contact US: help@patsnap.com