Cerebrovascular image segmentation method based on multi-attention dense connection generative adversarial network
A dense connection and image segmentation technology, applied in image analysis, image enhancement, image data processing, etc., can solve the problem of cerebral blood vessel shape, large grayscale difference, difficult to achieve cerebrovascular segmentation, difficult to achieve complete segmentation of cerebrovascular area, etc. problem, to achieve the effect of alleviating the serious class imbalance problem
- Summary
- Abstract
- Description
- Claims
- Application Information
AI Technical Summary
Problems solved by technology
Method used
Image
Examples
Embodiment Construction
[0034] In order to better understand the technical solutions of the present invention, the embodiments of the present invention will be further described below in conjunction with the accompanying drawings.
[0035] The present invention is a cerebrovascular MRA image segmentation method based on multi-attention dense connection generation confrontation network (MA-DenseGAN), and its overall network structure and algorithm framework are as follows figure 1 As shown, the specific implementation details of each part are as follows:
[0036] Step 1: Use segmentation generation network S to predict and generate high-quality cerebrovascular segmentation maps
[0037] According to the characteristics of cerebrovascular MRA images, the segmentation generation network S designs and optimizes the network structure to enhance the feature information extraction ability, information transmission ability and feature discrimination ability to improve the segmentation accuracy. The specific process...
PUM
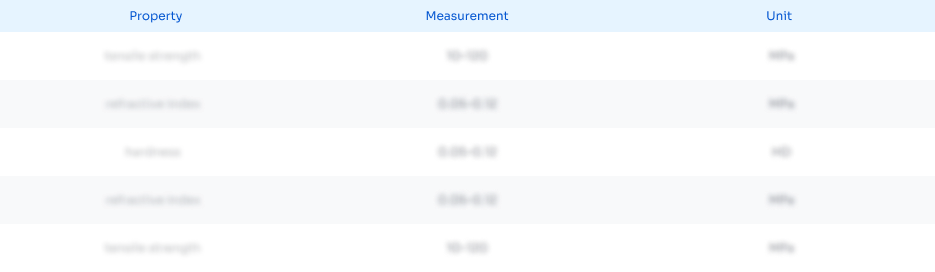
Abstract
Description
Claims
Application Information
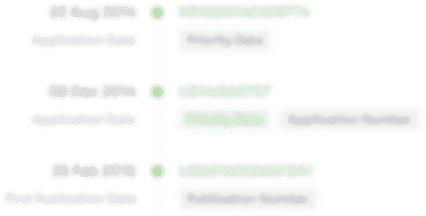
- R&D Engineer
- R&D Manager
- IP Professional
- Industry Leading Data Capabilities
- Powerful AI technology
- Patent DNA Extraction
Browse by: Latest US Patents, China's latest patents, Technical Efficacy Thesaurus, Application Domain, Technology Topic.
© 2024 PatSnap. All rights reserved.Legal|Privacy policy|Modern Slavery Act Transparency Statement|Sitemap