Entity relationship extraction method based on multi-convolution-window size attention convolutional neural network
A convolutional neural network and entity relationship technology, which is applied in the field of entity relationship extraction based on multi-convolution window size attention convolutional neural network, can solve the problems of error transmission, large labor and time cost, network performance degradation, etc. Avoid complicated feature engineering, improve accuracy, and run fast
- Summary
- Abstract
- Description
- Claims
- Application Information
AI Technical Summary
Problems solved by technology
Method used
Image
Examples
Embodiment Construction
[0038] The accompanying drawings are for illustrative purposes only and cannot be construed as limiting the patent;
[0039] In order to better illustrate this embodiment, some parts in the drawings will be omitted, enlarged or reduced, and do not represent the size of the actual product;
[0040] For those skilled in the art, it is understandable that some well-known structures and descriptions thereof may be omitted in the drawings.
[0041] The technical solutions of the present invention will be further described below in conjunction with the accompanying drawings and embodiments.
[0042] Such as figure 1 As shown, this application proposes a convolutional neural network entity relationship extraction method based on multi-convolution window size attention. The overall network structure is mainly divided into input layer, convolution pooling layer, multi-window size attention layer and full connection layer. First, convert each word in the input sentence into a word vect...
PUM
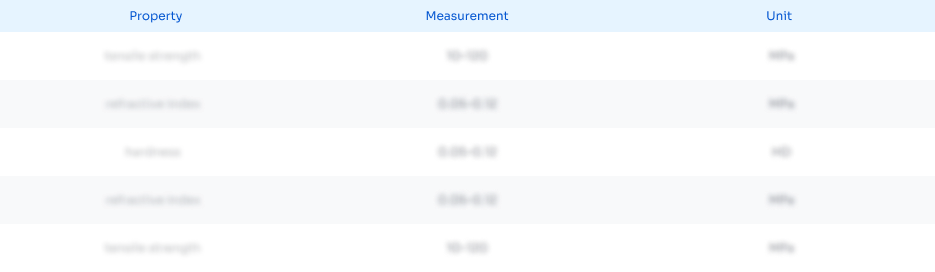
Abstract
Description
Claims
Application Information
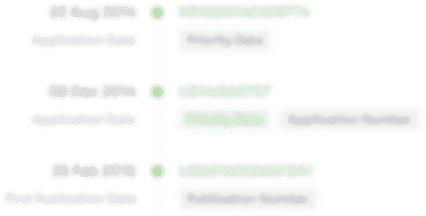
- R&D
- Intellectual Property
- Life Sciences
- Materials
- Tech Scout
- Unparalleled Data Quality
- Higher Quality Content
- 60% Fewer Hallucinations
Browse by: Latest US Patents, China's latest patents, Technical Efficacy Thesaurus, Application Domain, Technology Topic, Popular Technical Reports.
© 2025 PatSnap. All rights reserved.Legal|Privacy policy|Modern Slavery Act Transparency Statement|Sitemap|About US| Contact US: help@patsnap.com