Energy efficiency-oriented multi-agent deep reinforcement learning optimization method for unmanned aerial vehicle group
A technology of energy efficiency and reinforcement learning, which is applied in wireless communication, power management, electrical components, etc., can solve problems such as the inability of the algorithm to converge and converge, and achieve the effects of enhancing dynamic adaptability, improving life cycle, and improving energy efficiency
- Summary
- Abstract
- Description
- Claims
- Application Information
AI Technical Summary
Problems solved by technology
Method used
Image
Examples
Embodiment Construction
[0039] In order to make the purpose, technical solution and advantages of the present invention clearer, the present invention will be further described in detail below in conjunction with the accompanying drawings.
[0040] Such as image 3 As shown, the present invention discloses an energy efficiency-oriented UAV swarm multi-agent deep reinforcement learning optimization method, including the following steps:
[0041] S1. Obtain the current status information of the UAV cluster;
[0042] S2. Obtain the historical information of the UAV cluster, the historical information includes historical status information and historical decision information;
[0043] For each time slot, the historical information of multiple previous time slots is collected as the input of the neural network for learning, so as to obtain the decision information of the current time slot.
[0044]S3. Using the improved DQN deep reinforcement learning method based on Q-learning, using the historical inf...
PUM
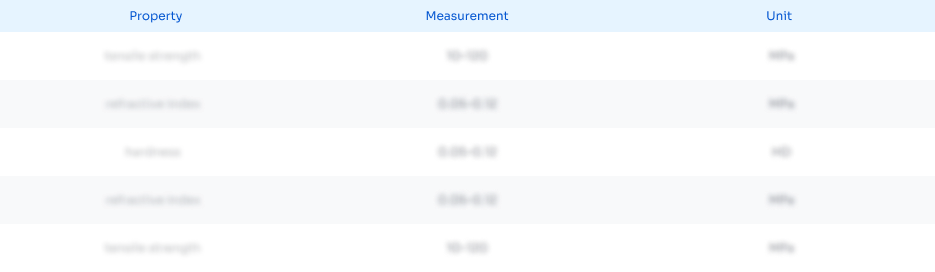
Abstract
Description
Claims
Application Information
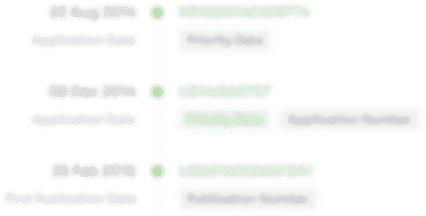
- R&D
- Intellectual Property
- Life Sciences
- Materials
- Tech Scout
- Unparalleled Data Quality
- Higher Quality Content
- 60% Fewer Hallucinations
Browse by: Latest US Patents, China's latest patents, Technical Efficacy Thesaurus, Application Domain, Technology Topic, Popular Technical Reports.
© 2025 PatSnap. All rights reserved.Legal|Privacy policy|Modern Slavery Act Transparency Statement|Sitemap|About US| Contact US: help@patsnap.com