Bayesian network inference method based on random computing theory
A technology of Bayesian network and reasoning method, which is applied in the field of Bayesian network reasoning based on stochastic computing theory, and can solve the problems of consuming hardware resources, slow calculation speed, and high power consumption of the system
- Summary
- Abstract
- Description
- Claims
- Application Information
AI Technical Summary
Problems solved by technology
Method used
Image
Examples
Embodiment Construction
[0023] The present invention will be further described below in conjunction with the accompanying drawings and embodiments.
[0024] According to the de Mouvre-Laplace theorem, when n is large enough, random numbers with normal distribution can also be obtained from the binomial distribution. The binomial distribution is a "0-1" sequence of length n. In the subsequent calculation , instead of using traditional computing operations, it uses a method based on random computing theory, directly allowing the "0-1" sequence to participate in the calculation. The calculation object of random calculation is the probability number represented by the bit stream. The value represented is only related to the length of the bit stream and the number of '1', and has nothing to do with the position. For example, 4 / 8 can be represented by an 8-bit bit stream 01101010 , so that only one AND gate is needed to realize the multiplication operation.
[0025] Such as figure 1 As shown, the Bayesia...
PUM
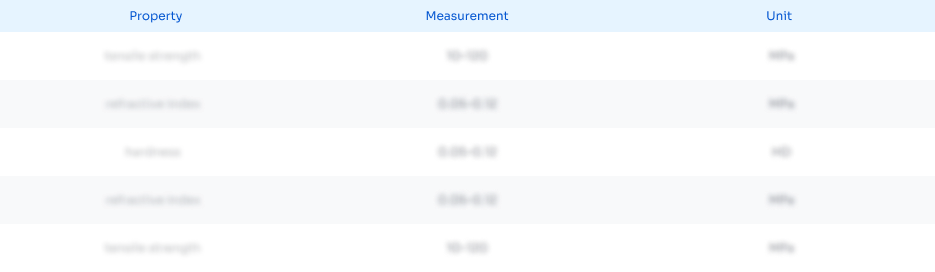
Abstract
Description
Claims
Application Information
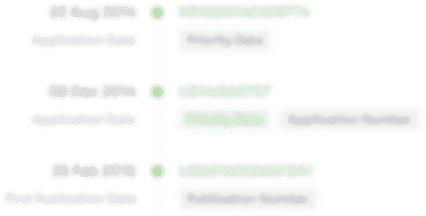
- R&D
- Intellectual Property
- Life Sciences
- Materials
- Tech Scout
- Unparalleled Data Quality
- Higher Quality Content
- 60% Fewer Hallucinations
Browse by: Latest US Patents, China's latest patents, Technical Efficacy Thesaurus, Application Domain, Technology Topic, Popular Technical Reports.
© 2025 PatSnap. All rights reserved.Legal|Privacy policy|Modern Slavery Act Transparency Statement|Sitemap|About US| Contact US: help@patsnap.com