Cross-domain three-dimensional model retrieval method based on multi-level feature alignment network
A three-dimensional model, feature pair technology, applied in digital data information retrieval, special data processing applications, image data processing and other directions, to achieve the effect of improving accuracy and retrieval accuracy
- Summary
- Abstract
- Description
- Claims
- Application Information
AI Technical Summary
Problems solved by technology
Method used
Image
Examples
Embodiment 1
[0031] A cross-domain 3D model retrieval method based on multi-level feature alignment network, see figure 1 , the method includes the following steps:
[0032] 101: First, use a virtual camera to take virtual photos of the models in the 3D model database to generate multi-view data;
[0033] 102: A new network framework is designed: a multi-level feature alignment network for feature alignment of images and 3D models at the domain level and class level.
[0034] Among them, the feature extraction part of the multi-level feature alignment network adopts the classic CNN network (AlexNet, etc.) as the basic network structure. When extracting image features and 3D model multi-view features, the two parts of the CNN network share parameters, and then adopt a pooling layer to integrate the features of all views in the 3D model multi-view into a 3D descriptor as the feature representation of the 3D model.
[0035] 103: Multi-level feature alignment network. In order to better extr...
Embodiment 2
[0039] The scheme in embodiment 1 is further introduced below in conjunction with specific examples and calculation formulas, see the following description for details:
[0040] 201: First use the virtual camera to take virtual photos of the models in the 3D model database to generate multi-view data;
[0041] Wherein, the above step 201 mainly includes:
[0042] A set of viewpoints is predefined, and the viewpoints are the viewpoints for observing the target object. Let M be the number of predefined viewpoints. In the embodiment of the present invention, M is taken as 12, that is, a virtual For the camera, the viewpoints are completely evenly distributed around the target object. By picking different interval angles, different sets of views of the model can be obtained.
[0043] All the objects in the 3D model database are projected to obtain a set of views for each target, and a set of views of all targets constitutes a multi-view model database.
[0044] 202: Design a ne...
Embodiment 3
[0065] Below in conjunction with concrete test, the scheme in embodiment 1 and 2 is carried out feasibility verification, see the following description for details:
[0066] Using six commonly used evaluation indicators in 3D models [9] : NN, FT, ST, F, DCG, ANMMR to measure the performance of this method.
[0067] The implementation methods of these six evaluation criteria are as follows:
[0068] NN: Calculate the percentage of the nearest matching model that belongs to the query category;
[0069] FT: Calculate the recall rate of the first K relevant matching samples, where K is the cardinality of the query category;
[0070] ST: Calculate the recall rate of the first 2K relevant matching samples;
[0071] F: Comprehensive measurement of precision and recall for a certain number of retrieval results;
[0072] DCG: assign higher weights to 3D models related to the query image and lower weights to irrelevant 3D models, and then perform statistical measurements;
[0073] ...
PUM
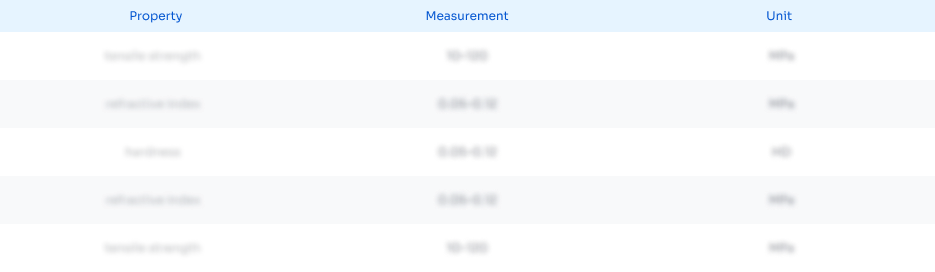
Abstract
Description
Claims
Application Information
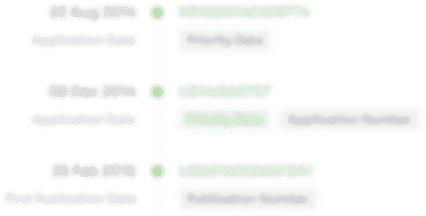
- R&D Engineer
- R&D Manager
- IP Professional
- Industry Leading Data Capabilities
- Powerful AI technology
- Patent DNA Extraction
Browse by: Latest US Patents, China's latest patents, Technical Efficacy Thesaurus, Application Domain, Technology Topic.
© 2024 PatSnap. All rights reserved.Legal|Privacy policy|Modern Slavery Act Transparency Statement|Sitemap