Video action classification and recognition method based on double-flow collaborative network
A collaborative network, classification and recognition technology, applied in character and pattern recognition, instruments, computer parts, etc., can solve problems such as missing key technologies, inability to end-to-end, processing, etc.
- Summary
- Abstract
- Description
- Claims
- Application Information
AI Technical Summary
Problems solved by technology
Method used
Image
Examples
Embodiment 1
[0052] A video action classification and recognition method based on a dual-stream collaborative network, combining figure 1 , figure 2 and image 3 As shown, firstly, the spatial sequence feature is extracted from the video frame and the time domain sequence feature is extracted from the video optical flow field through the convolutional network. The expression of the spatial sequence feature is The characteristic expression of the time domain sequence is in d is the dimension of the feature; then construct a connection unit to allow the heterogeneous spatial sequence features and time domain sequence features to exchange information; then construct a shared unit to perform the fusion of the fused spatial sequence features and the fused time domain sequence features respectively Sequential feature aggregation to obtain aggregated spatial features and aggregated time domain features; the aggregated spatial feature expression is Z f , the time-domain feature expression ...
Embodiment 2
[0062] On the basis of above-mentioned embodiment 1, in order to realize the present invention better, combine figure 1 , figure 2 As shown, further, a shared unit is constructed to perform sequential feature aggregation on the fused spatial sequence features and the fused time domain sequence features; the fused spatial sequence features are aggregated into the spatial feature Z f , the fused time-domain sequence features are aggregated into a time-domain feature Z o ; The time domain feature Z o and the spatial feature Z f At the same time, regularization is performed, and then the shared weight layer is input, and then the temporal feature classification score and the spatial feature classification score are extracted; finally, the temporal feature classification score and the spatial feature classification score are fused into predicted spatiotemporal features for actual video action recognition Classification score vector; the predicted spatiotemporal feature classifi...
Embodiment 3
[0065] On the basis of any one of the above-mentioned embodiments 1-2, in order to better realize the present invention, further, select a sample set for training to generate a classifier model that includes correct spatio-temporal feature classification scores for action classification; use cross-entropy loss function, heterogeneous triplet pair loss function, discriminative embedding-limited loss function combined function as the loss function for training.
[0066] Working principle: Select a sample set for pre-training, train a classifier model, introduce a combination of cross-entropy loss function, heterogeneous triplet pair loss function, and discriminative embedding limit loss function as the training loss function, which can make the classification obtained by pre-training The device model is more realistic and reliable, and the classification is more aggregated.
[0067] Other parts of this embodiment are the same as those of any one of Embodiments 1-2 above, so deta...
PUM
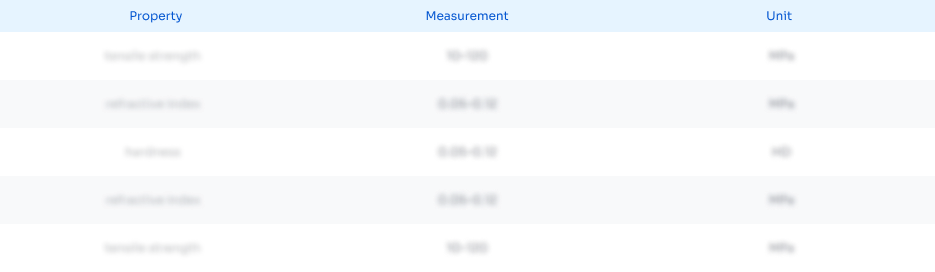
Abstract
Description
Claims
Application Information
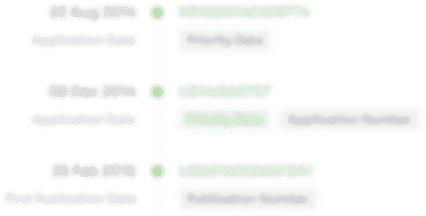
- R&D Engineer
- R&D Manager
- IP Professional
- Industry Leading Data Capabilities
- Powerful AI technology
- Patent DNA Extraction
Browse by: Latest US Patents, China's latest patents, Technical Efficacy Thesaurus, Application Domain, Technology Topic, Popular Technical Reports.
© 2024 PatSnap. All rights reserved.Legal|Privacy policy|Modern Slavery Act Transparency Statement|Sitemap|About US| Contact US: help@patsnap.com