Power load prediction method based on EEMD secondary decomposition
A forecasting method and electric load technology, applied in forecasting, instruments, biological neural network models, etc., can solve problems such as irregularities and large fluctuations in high-frequency sub-sequences, and achieve high-precision forecasting, improve forecasting accuracy, and good short-term load The effect of predictive power
- Summary
- Abstract
- Description
- Claims
- Application Information
AI Technical Summary
Problems solved by technology
Method used
Image
Examples
Embodiment 1
[0039] This embodiment 1 provides a power load forecasting method based on EEMD secondary decomposition, and its steps are as follows:
[0040] Step 1: Build a load time series: collect a large industrial user from May 4, 2018 to June 11, 2018, June 13, 2018 to June 18, 2018, June 21, 2018 to 2018 Power load sampling data for three time periods on July 15 was collected at 30s intervals to construct a time series.
[0041] Step two, data preprocessing: null values are eliminated, data is absoluteized, and historical load data is normalized. The formula is:
[0042] among them Represents the normalized load value at time i, x i Represents the load value at time i, x min And x max Respectively represent the minimum load value and the maximum load value in the time series.
[0043] Step three, a signal decomposition: add a Gaussian noise sequence with an amplitude of 0.05 to the time series, and then perform EMD decomposition of the time series after adding noise to obtain the intrin...
PUM
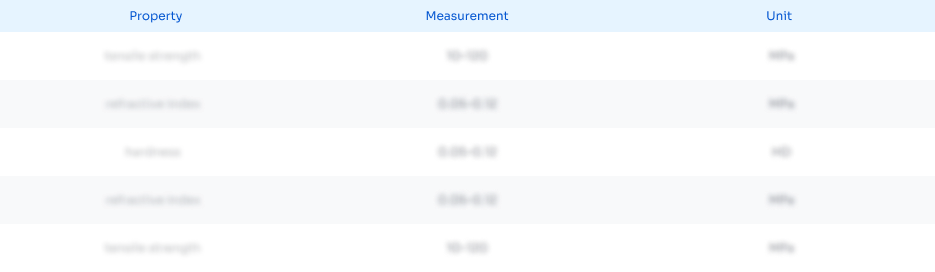
Abstract
Description
Claims
Application Information
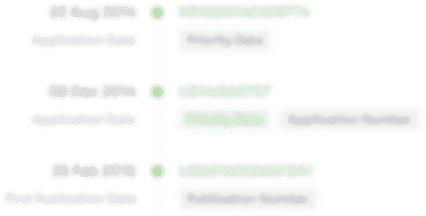
- R&D
- Intellectual Property
- Life Sciences
- Materials
- Tech Scout
- Unparalleled Data Quality
- Higher Quality Content
- 60% Fewer Hallucinations
Browse by: Latest US Patents, China's latest patents, Technical Efficacy Thesaurus, Application Domain, Technology Topic, Popular Technical Reports.
© 2025 PatSnap. All rights reserved.Legal|Privacy policy|Modern Slavery Act Transparency Statement|Sitemap|About US| Contact US: help@patsnap.com