Graph model text abstract generation method based on word frequency and semantics
A graph model and text technology, applied in the field of text summarization, can solve problems such as impracticality, long-distance dependence, and learning frameworks that cannot accurately obtain sentence semantic information, so as to save time and improve pertinence
- Summary
- Abstract
- Description
- Claims
- Application Information
AI Technical Summary
Problems solved by technology
Method used
Image
Examples
Embodiment Construction
[0065] 1. Word segmentation: First, each sentence is segmented and part-of-speech tagged, using the standard word segmenter in the natural language processing package Hanlp. Using the principle of shortest path word segmentation (Viterbi algorithm is used to solve the shortest path) and HMM (hidden Markov model), realize text word segmentation and part-of-speech tagging. It also realizes the recognition of entity names such as numbers, person names, place names, and organization names, and in order to improve the pertinence of word segmentation in specific fields, a user dictionary function is further added to the word segmentation module.
[0066] 2. Filter terms: remove stop words, and perform noise reduction processing on the text. The system filters terms in units of sentences. The first is stop word filtering, low-frequency word filtering, and part-of-speech filtering.
[0067] 3. Word vector training: The BM25 algorithm uses word frequency information to represent the wo...
PUM
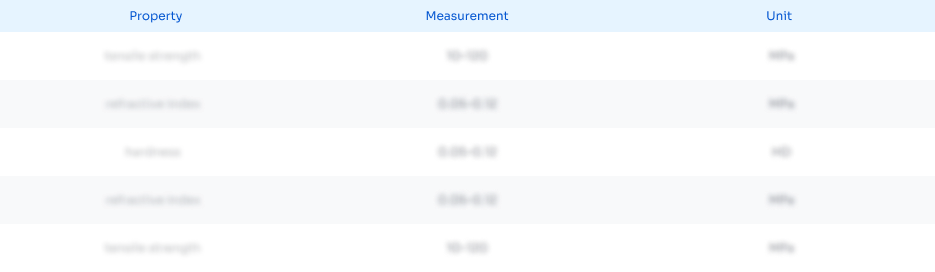
Abstract
Description
Claims
Application Information
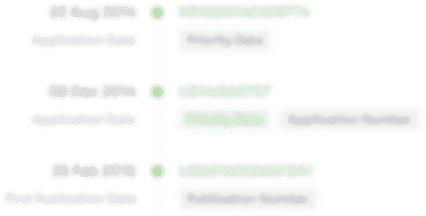
- R&D Engineer
- R&D Manager
- IP Professional
- Industry Leading Data Capabilities
- Powerful AI technology
- Patent DNA Extraction
Browse by: Latest US Patents, China's latest patents, Technical Efficacy Thesaurus, Application Domain, Technology Topic, Popular Technical Reports.
© 2024 PatSnap. All rights reserved.Legal|Privacy policy|Modern Slavery Act Transparency Statement|Sitemap|About US| Contact US: help@patsnap.com