Indoor home automatic layout algorithm for detecting empty room features based on deep learning
A technology of deep learning and feature detection, applied in neural learning methods, biological neural network models, neural architectures, etc., can solve problems such as lack of furniture
- Summary
- Abstract
- Description
- Claims
- Application Information
AI Technical Summary
Problems solved by technology
Method used
Image
Examples
Embodiment Construction
[0035] The object detection model generally solves the problem of given the existence of objects in the picture, and then requires the model to output the boundary of the object type. The algorithm of the present invention applies the object detection model to non-object detection problems. The detected object does not exist in the input image, and there are only empty rooms in the input image. The experiment found that by detecting the unique model of the door, window and wall, the object detection model can be used to predict the type, size and position of the furniture.
[0036] Step 1, generate an image of an empty room. Based on the OBJ file of the floor plan, a 3-channel empty room picture containing only the picture information of the door, window and wall is generated, and the door, window and wall are represented by different colors.
[0037] Step 2, organize the data labels. Quantify the information such as the type of furniture, the size of the furniture, and the...
PUM
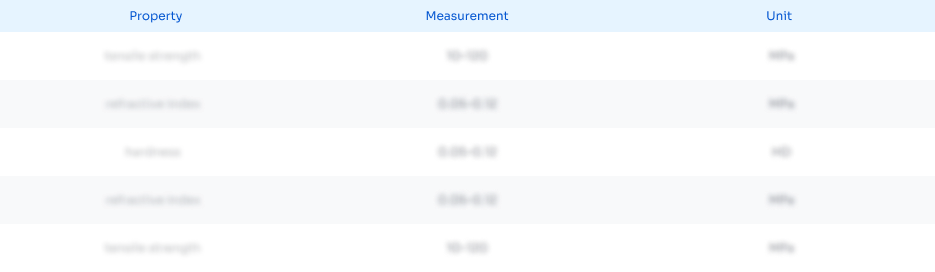
Abstract
Description
Claims
Application Information
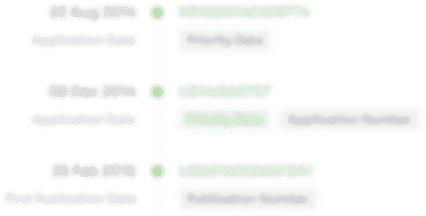
- R&D Engineer
- R&D Manager
- IP Professional
- Industry Leading Data Capabilities
- Powerful AI technology
- Patent DNA Extraction
Browse by: Latest US Patents, China's latest patents, Technical Efficacy Thesaurus, Application Domain, Technology Topic.
© 2024 PatSnap. All rights reserved.Legal|Privacy policy|Modern Slavery Act Transparency Statement|Sitemap