Safe semi-supervised learning electroencephalogram signal identification method based on self-adaptive risk degree
A semi-supervised learning and EEG signal technology, applied in the fields of application, medical science, electrical digital data processing, etc., can solve the problem of lack of safe semi-supervised signal recognition methods, achieve enhanced robustness and practicability, and improve recognition rate , the effect of reducing risk
- Summary
- Abstract
- Description
- Claims
- Application Information
AI Technical Summary
Problems solved by technology
Method used
Image
Examples
Embodiment Construction
[0014] The present invention is further illustrated in conjunction with the accompanying drawings. It should be understood that these embodiments are only used to illustrate the present invention and are not intended to limit the scope of the present invention. After reading the present invention, those skilled in the art will modify various equivalent forms of the present invention All fall within the scope defined by the appended claims of this application.
[0015] Such as figure 1 As shown, the implementation of the present invention mainly includes four steps: (1) input and preprocessing of EEG signals to form a database; (2) construct a neighbor graph W, and train a multi-class supervised classifier to obtain predictions of unlabeled samples label; (3) using l 2,1 The norm constructs the optimization problem, obtains the objective function, and trains the safe semi-supervised classifier through iterative optimization; (4) Input the signal to be identified, and use the s...
PUM
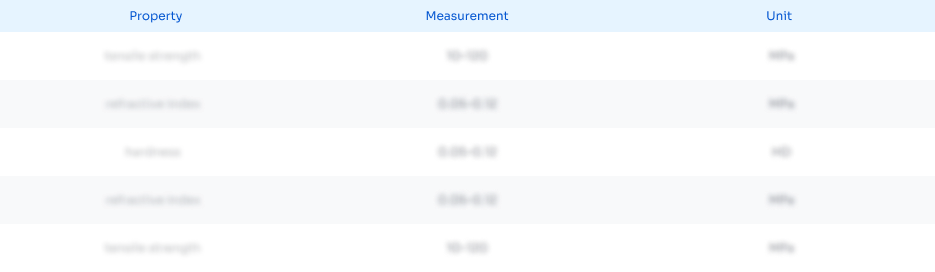
Abstract
Description
Claims
Application Information
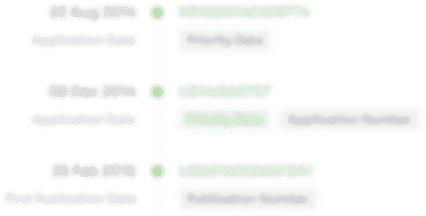
- R&D Engineer
- R&D Manager
- IP Professional
- Industry Leading Data Capabilities
- Powerful AI technology
- Patent DNA Extraction
Browse by: Latest US Patents, China's latest patents, Technical Efficacy Thesaurus, Application Domain, Technology Topic, Popular Technical Reports.
© 2024 PatSnap. All rights reserved.Legal|Privacy policy|Modern Slavery Act Transparency Statement|Sitemap|About US| Contact US: help@patsnap.com