Relation prediction method combining logic rules and fragmented knowledge
A prediction method and a fragmented technology, applied in knowledge expression, reasoning methods, semantic analysis, etc., can solve the problems of not being able to fully utilize the value of fragmented knowledge and accurately expressing semantic relationships
- Summary
- Abstract
- Description
- Claims
- Application Information
AI Technical Summary
Problems solved by technology
Method used
Image
Examples
Embodiment Construction
[0056] The technical solution of the present invention will be specifically described below in conjunction with the accompanying drawings.
[0057] The present invention provides a relationship prediction method combining logical rules and fragmented knowledge. Firstly, the fact triples and logical rules are unifiedly modeled, and the hidden semantic information is embedded into the relational reasoning model based on knowledge representation; secondly , combined with fragmented knowledge, iteratively updated, making the knowledge base more complete. The specific implementation of this method is as follows:
[0058] The first stage: Model the direct fact triples in the knowledge base to obtain the vector representations of all entities and relationships in the knowledge base, which are used in the third stage to calculate the semantic relevance between rules;
[0059] The second stage: mining a set of logical rules that can represent the semantic information of the knowledge ...
PUM
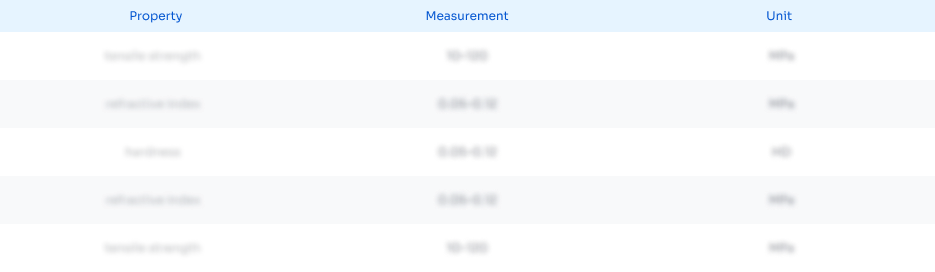
Abstract
Description
Claims
Application Information
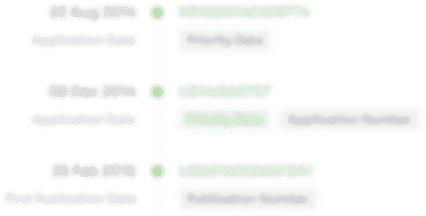
- R&D
- Intellectual Property
- Life Sciences
- Materials
- Tech Scout
- Unparalleled Data Quality
- Higher Quality Content
- 60% Fewer Hallucinations
Browse by: Latest US Patents, China's latest patents, Technical Efficacy Thesaurus, Application Domain, Technology Topic, Popular Technical Reports.
© 2025 PatSnap. All rights reserved.Legal|Privacy policy|Modern Slavery Act Transparency Statement|Sitemap|About US| Contact US: help@patsnap.com