Interactive data extension method based on Poisson image fusion and image stylization
An interactive data and Poisson image technology, applied in image data processing, image enhancement, image analysis, etc., can solve the problems of uncontrollable output, difficult training of GANs model, and limited use, so as to achieve excellent performance and improve model generalization effect of ability
- Summary
- Abstract
- Description
- Claims
- Application Information
AI Technical Summary
Problems solved by technology
Method used
Image
Examples
Embodiment 1
[0047] An interactive data augmentation method based on Poisson image fusion, see figure 1 , the method includes the following steps:
[0048] 1. Interactive Basic Framework
[0049] Interactive data augmentation process see figure 1 , see the interactive interface figure 2 , before the interactive program runs, the defect library construction program should be run first. For the original image with json annotation information, by reading the annotation information, the defect is extracted from the original image and saved as a defect image containing only defect information, and the supporting json annotation information of the defect information is automatically generated, and the All defects are stored in corresponding folders by category to build a defect information library.
[0050] At the same time, an event function is added to the interactive interface to obtain the defect type and the coordinates of the defect generation location that the user wants to generate ...
Embodiment 2
[0086] Combine below image 3 Carry out feasibility verification to the scheme in embodiment 1, see the following description for details:
[0087] according to figure 1 The flow structure shown builds the interactive framework in the embodiment of the present invention, and obtains the defect generation location and defect type selected by the user in real time. And prepare the training data set for the style transfer model and train the style transfer model, and perform Poisson correction on the edge area of the fusion defect image to eliminate the fusion trace.
[0088] From image 3 , it can be seen that the data expansion results obtained in the embodiment of the present invention have no trace of fusion, and the sample availability is high. image 3 Among them, the first column is the original image, the second column is the image directly fused without stylization and Poisson correction, the third column is the image generated by the example of the present inventio...
PUM
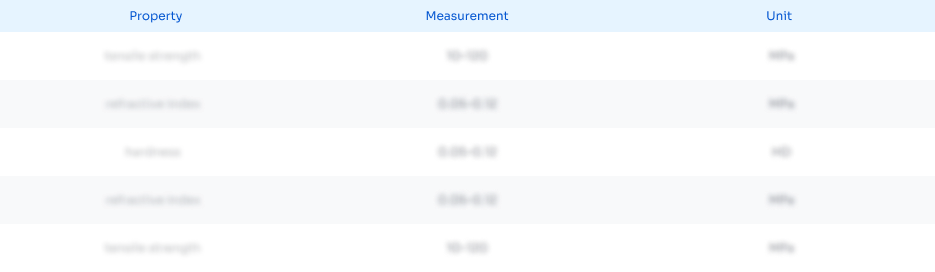
Abstract
Description
Claims
Application Information
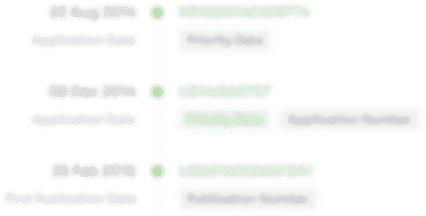
- R&D Engineer
- R&D Manager
- IP Professional
- Industry Leading Data Capabilities
- Powerful AI technology
- Patent DNA Extraction
Browse by: Latest US Patents, China's latest patents, Technical Efficacy Thesaurus, Application Domain, Technology Topic, Popular Technical Reports.
© 2024 PatSnap. All rights reserved.Legal|Privacy policy|Modern Slavery Act Transparency Statement|Sitemap|About US| Contact US: help@patsnap.com