Video recommendation method based on multimodal video content and multi-task learning
A technology of multi-task learning and video content, applied in the field of video recommendation based on multi-modal video content and multi-task learning, can solve the problems of dependence, cold start, video inaccuracy, etc., and achieve the effect of reducing the scale of parameters
- Summary
- Abstract
- Description
- Claims
- Application Information
AI Technical Summary
Problems solved by technology
Method used
Image
Examples
Embodiment
[0042] figure 1 The flow chart of the video recommendation method based on multi-modal video content and multi-task learning disclosed in the present invention is given, which specifically includes the following steps:
[0043] T1, video multi-modal feature extraction:
[0044] a. The extraction of video frames, through the opencv video reading class cv2.VideoCapture to intercept video frame pictures, save them in the path folder, the number of frames starts from 0, considering the short and precise characteristics of short videos, intercept each frame of video The picture is intercepted without skipping frames.
[0045] b. Video static feature extraction, adjust the size of each frame of the video to [299, 299] and then input it into the pre-trained Inception-V3 network, as attached figure 1 As shown, the input is mapped to a 2048-dimensional feature vector as the static original feature vector of the video frame. In order to preserve the information of each frame of the vide...
PUM
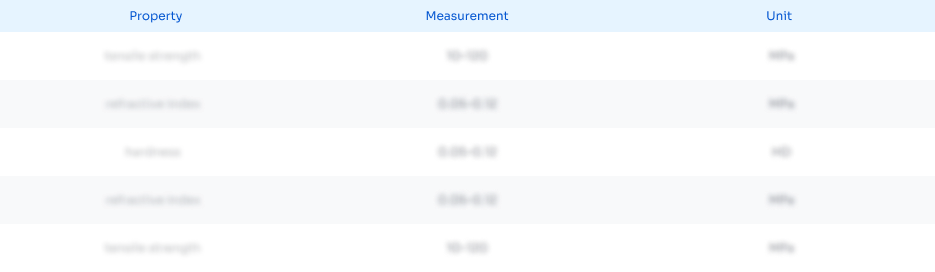
Abstract
Description
Claims
Application Information
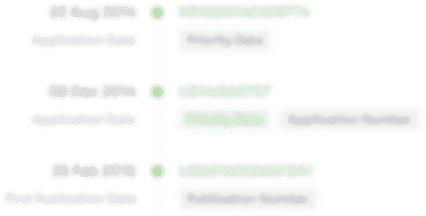
- R&D Engineer
- R&D Manager
- IP Professional
- Industry Leading Data Capabilities
- Powerful AI technology
- Patent DNA Extraction
Browse by: Latest US Patents, China's latest patents, Technical Efficacy Thesaurus, Application Domain, Technology Topic, Popular Technical Reports.
© 2024 PatSnap. All rights reserved.Legal|Privacy policy|Modern Slavery Act Transparency Statement|Sitemap|About US| Contact US: help@patsnap.com