Text rhythm prediction method based on multi-task multi-level model
A prediction method and multi-level technology, applied in neural learning methods, biological neural network models, instruments, etc., can solve problems such as long sentences without prosodic phrase boundaries and intonation phrase boundaries, troublesome training and parameter adjustment, and prosodic information errors. Achieve the effects of reducing long sentences without rhythmic pauses, optimizing bad problems, and improving information utilization
- Summary
- Abstract
- Description
- Claims
- Application Information
AI Technical Summary
Problems solved by technology
Method used
Image
Examples
Embodiment 1
[0031] Example 1, see Figure 1. This figure mainly explains the data processing, data encoding and model training. The specific implementation includes the following parts:
[0032] Step 101: Obtain training text, including common text regularization methods such as text length cropping, illegal characters and punctuation mark correction;
[0033] Step 102: In prosodic acoustics, full stops, question marks, exclamation marks, and commas are used as intonation phrase boundaries, and accordingly, commas, full stops, exclamation marks, question marks, and semicolons in the text are randomly removed, which can form long pauses in the text rhythm. Punctuation mark, the punctuation mark position is regarded as the intonation phrase level boundary point, and such text is used as the extended text as the training data; this step also includes the splicing of two or more short texts, which is used as the extended data for prosodic text training;
[0034] Step 103: use the character-lev...
Embodiment 2
[0037] Embodiment 2, see Figure 2, this figure is mainly the multi-task neural network model architecture part, the specific implementation includes the following parts, for the sake of clarity and conciseness, the description of the known functions and structures is omitted in the following description, and only the core points are explained :
[0038] As shown in the figure, firstly, after the input sentence enters the model, it is encoded, including word information and position information encoding. The methods used include but are not limited to common one-hot vectors, relative position encoding of trigonometric functions, etc.;
[0039] Using a multi-layer multi-head self-attention layer to extract text semantic analysis and prosodic structure information, the attention weight algorithm of the multi-head self-attention layer is not limited here;
[0040] Among them, the multi-layer self-attention model in the figure can be pre-trained using a large text corpus, or a mode...
Embodiment 3
[0045] Example 3, as shown in Figure 3, mainly explains the solution mechanism for the long sentences in the prediction stage that do not predict the boundaries of L2 and L3, that is, to generate a boundary based on the best, specifically:
[0046] As shown in the figure, it is assumed that after argmax is calculated by the output probability matrix of the L2 layer, all of them are O tags, that is, there are no prosodic phrase boundaries in the sentence, that is, only prosodic word boundaries. is for longer sentences;
[0047] Then adopt a more reasonable mechanism: make a slice from the B label, get the possible probability of the B label in all words, and select the position with the highest probability as the B label position.
[0048] Example 3, see Figure 3, describes the whole process of forecasting, specifically:
[0049] Step 401: Obtain predicted text;
[0050] Step 402: Carry out character-level encoding for the word table of the text to be predicted, similar to st...
PUM
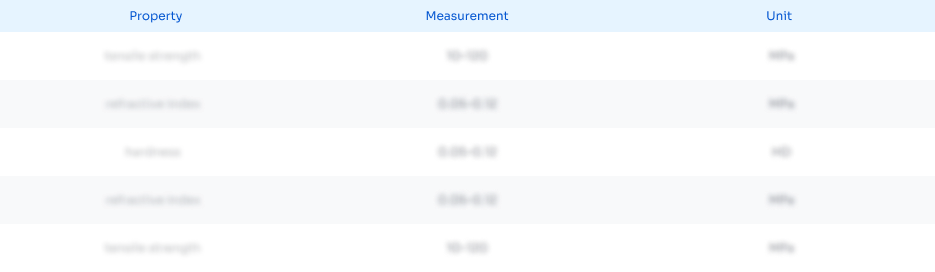
Abstract
Description
Claims
Application Information
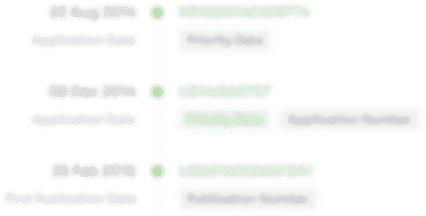
- Generate Ideas
- Intellectual Property
- Life Sciences
- Materials
- Tech Scout
- Unparalleled Data Quality
- Higher Quality Content
- 60% Fewer Hallucinations
Browse by: Latest US Patents, China's latest patents, Technical Efficacy Thesaurus, Application Domain, Technology Topic, Popular Technical Reports.
© 2025 PatSnap. All rights reserved.Legal|Privacy policy|Modern Slavery Act Transparency Statement|Sitemap|About US| Contact US: help@patsnap.com