Feature layer fusion method and device based on graph embedding canonical correlation analysis
A typical correlation analysis and fusion method technology, applied in the field of feature layer fusion, can solve problems such as poor recognition effect, achieve good recognition effect, improve feature discrimination, and feature fusion effect
- Summary
- Abstract
- Description
- Claims
- Application Information
AI Technical Summary
Problems solved by technology
Method used
Image
Examples
Embodiment 1
[0065] see figure 1 , this embodiment provides a feature layer fusion method based on graph embedding canonical correlation analysis, which can be applied in speech recognition, emotion recognition, medical image analysis, and multimedia event detection. In this embodiment, the feature layer fusion method is mainly divided into two stages. The first stage selects representative representations of multiple single-mode features, and the second stage establishes correlations between multi-mode features.
[0066] In this embodiment, it is assumed that the j-th sample of the i-th modality that needs to be fused is n is the number of samples per modality, i.e. the sample size. P represents the number of heterogeneous sample sets that need to be fused, di represents the i-th modality sample dimension, and c is the number of categories. for any vector For a matrix A∈R d×n , the i-th row of A is denoted as a i , column j is denoted as a j , so Wherein, the feature layer fus...
Embodiment 2
[0122] This embodiment provides a feature layer fusion method based on graph embedding canonical correlation analysis, and a simulation experiment is performed on the basis of Embodiment 1. In the experimental part of this embodiment, several sets of experiments are proposed to verify the effectiveness of the feature layer fusion method. These groups of experiments are combining multiple features extracted in a single modality and combining features extracted separately in different modalities. Among them, the experiment is divided into three parts. Parts A and B combine the experimental results of different feature vectors extracted by a single mode, respectively using the multi-feature handwriting data set in the UCI data set and the Hong Kong Polytechnic University finger vein database. Part C is the experimental results of combining different biological characteristics of the hand, using the finger vein database provided by the USM database, the palmprint public database a...
Embodiment 3
[0190] This embodiment provides a feature layer fusion device based on graph embedding typical correlation analysis, which applies the feature layer fusion method based on graph embedding typical correlation analysis of embodiment 1 or embodiment 2, and includes a feature selection module, a similar graph matrix Building blocks as well as fusion modules.
[0191] The feature selection module is used to map the samples in all modalities to the projection matrix of the space of the same classification result, and apply L21 norm regularization to the projection matrix to select independent complementary features in multiple unimodal feature spaces at the same time Among them, the feature selection module includes an initial objective function establishment unit, an optimization unit and an update unit. The initial objective function establishing unit is used to establish the initial objective function. The initial objective function is used to learn the projection matrices for v...
PUM
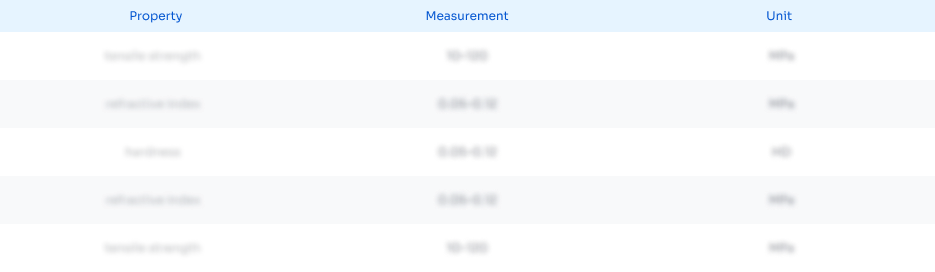
Abstract
Description
Claims
Application Information
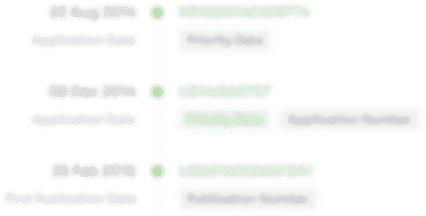
- R&D Engineer
- R&D Manager
- IP Professional
- Industry Leading Data Capabilities
- Powerful AI technology
- Patent DNA Extraction
Browse by: Latest US Patents, China's latest patents, Technical Efficacy Thesaurus, Application Domain, Technology Topic, Popular Technical Reports.
© 2024 PatSnap. All rights reserved.Legal|Privacy policy|Modern Slavery Act Transparency Statement|Sitemap|About US| Contact US: help@patsnap.com