Scene image labeling method based on coarse-fine granularity multi-image multi-label learning
A scene image and multi-label technology, which is applied in the direction of graphic image conversion, image analysis, image data processing, etc., can solve problems that cannot be directly applied, and there is no MIML learning model feature example vector
- Summary
- Abstract
- Description
- Claims
- Application Information
AI Technical Summary
Problems solved by technology
Method used
Image
Examples
Embodiment Construction
[0050] The specific implementation manners of the present invention will be further described in detail below in conjunction with the accompanying drawings and embodiments. The following examples are used to illustrate the present invention, but are not intended to limit the scope of the present invention.
[0051] In this embodiment, the scene image labeling method based on coarse-fine-grained multi-image multi-label learning, such as figure 1 shown, including the following steps:
[0052] Step 1: Obtain the original scene image dataset and the corresponding label set;
[0053] In the embodiment of the present invention, the real image data set: PASCAL VISUAL Object Challenge 2012 data set (VOC12) is used as the original scene graph data set; the data set has a total of 1073 images, and each image has corresponding multiple objects, each Objects have a label. The dataset includes a total of 20 types of objects, such as "car", "boat", "dog", "human", "sheep" and "chair".
...
PUM
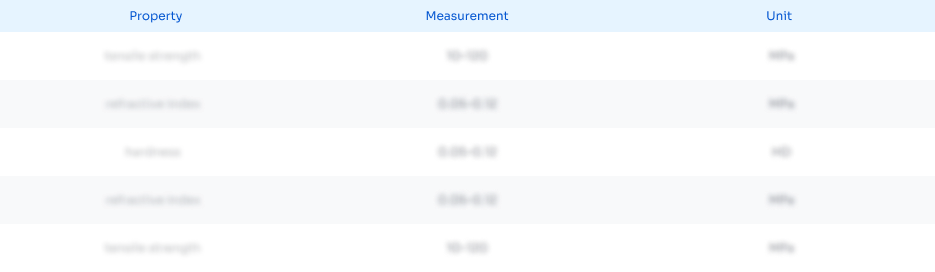
Abstract
Description
Claims
Application Information
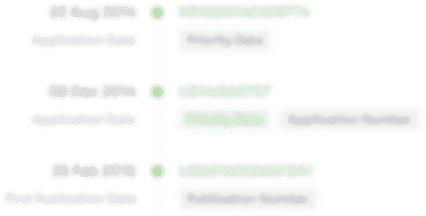
- R&D
- Intellectual Property
- Life Sciences
- Materials
- Tech Scout
- Unparalleled Data Quality
- Higher Quality Content
- 60% Fewer Hallucinations
Browse by: Latest US Patents, China's latest patents, Technical Efficacy Thesaurus, Application Domain, Technology Topic, Popular Technical Reports.
© 2025 PatSnap. All rights reserved.Legal|Privacy policy|Modern Slavery Act Transparency Statement|Sitemap|About US| Contact US: help@patsnap.com