Super-resolution reconstruction method and system for image
A technology of super-resolution reconstruction and image reconstruction, applied in the field of computer vision, can solve the problem of poor super-resolution effect by using and fusing different layers of feature information, and achieve the effect of good feature representation, alleviation of performance bottlenecks, and good feature representation ability.
- Summary
- Abstract
- Description
- Claims
- Application Information
AI Technical Summary
Problems solved by technology
Method used
Image
Examples
Embodiment 1
[0056] An image super-resolution reconstruction method, such as figure 1 shown, including the following steps:
[0057] S1. Dividing the low-resolution image to be processed into blocks to obtain multiple image blocks;
[0058] Specifically, in this embodiment, the low-resolution image to be processed is sequentially and repeatedly intercepted image blocks with a size of H×W in a sliding window manner. Specifically, the low-resolution RGB image to be processed has repeated image blocks with a size of H×W cut from left to right and from top to bottom in the form of a sliding window.
[0059] S2. Based on the channel attention mechanism and position attention mechanism of the image, the features on each image block are extracted at different depths, and the feature maps of different depths corresponding to each image block are obtained;
[0060] Preferably, the following steps are included:
[0061] S21. Using a convolutional neural network to extract shallow features of the ...
Embodiment 2
[0089] An image super-resolution system, such as image 3 As shown, including: image interception module 1, feature extraction module 2, image reconstruction module 3 and image recombination module 4;
[0090] Wherein, the image interception module 1 is used for dividing the low-resolution image to be processed into blocks to obtain a plurality of image blocks, and output them to the feature extraction module 2;
[0091] The feature extraction module 2 is used for image-based channel attention mechanism and position attention mechanism, extracts the features of each image block input by the image interception module at different depths, obtains feature maps of different depths corresponding to each image block, and outputs to In the image reconstruction module 3; preferably, the feature extraction module includes a shallow network unit 21 and a deep network unit 22; wherein the shallow network unit 21 is used to extract the shallow features of the image block using a convoluti...
Embodiment 3
[0098] A storage medium, when a computer reads the instructions, it causes the computer to execute the image super-resolution reconstruction method provided in Embodiment 1 of the present invention.
PUM
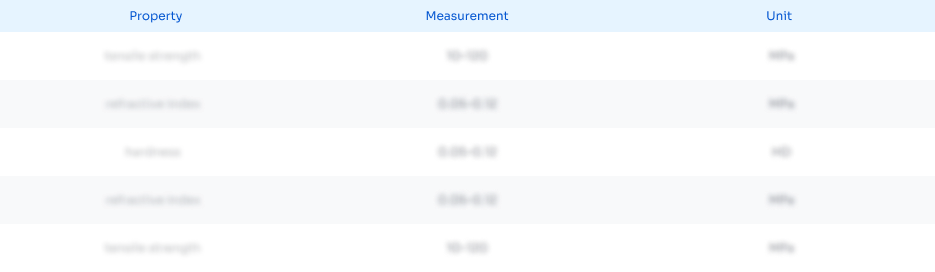
Abstract
Description
Claims
Application Information
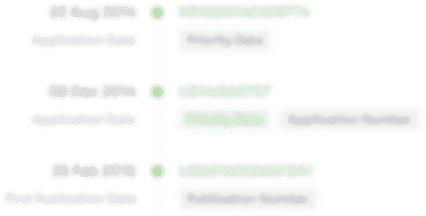
- R&D Engineer
- R&D Manager
- IP Professional
- Industry Leading Data Capabilities
- Powerful AI technology
- Patent DNA Extraction
Browse by: Latest US Patents, China's latest patents, Technical Efficacy Thesaurus, Application Domain, Technology Topic, Popular Technical Reports.
© 2024 PatSnap. All rights reserved.Legal|Privacy policy|Modern Slavery Act Transparency Statement|Sitemap|About US| Contact US: help@patsnap.com