Few-sample pedestrian re-identification method based on deep multi-example learning
A multi-example learning and pedestrian re-recognition technology, applied in character and pattern recognition, instruments, biological neural network models, etc., can solve problems such as difficulty in marking pedestrian pictures, and achieve good recognition accuracy.
- Summary
- Abstract
- Description
- Claims
- Application Information
AI Technical Summary
Problems solved by technology
Method used
Image
Examples
Embodiment Construction
[0038] Cooperate see figure 1 , figure 2 As shown, the present invention uses a multi-instance and few-sample pedestrian feature learning network framework to perform feature learning and identity recognition on pedestrian pictures. The multi-instance and few-sample pedestrian feature learning network framework mainly includes three stages: network pre-training stage, data set expansion stage, and network fine-tuning stage; it includes two networks: feature extraction sub-network and feature aggregation network.
[0039] In the network pre-training stage, a large amount of labeled training data in the original domain is used to train and learn the feature extraction sub-network, and the learned parameters are used as initial parameters for the feature extraction sub-network to apply to the target domain.
[0040] The data set expansion stage and the network fine-tuning stage are iteratively carried out until the two networks reach convergence, then the feature extraction sub...
PUM
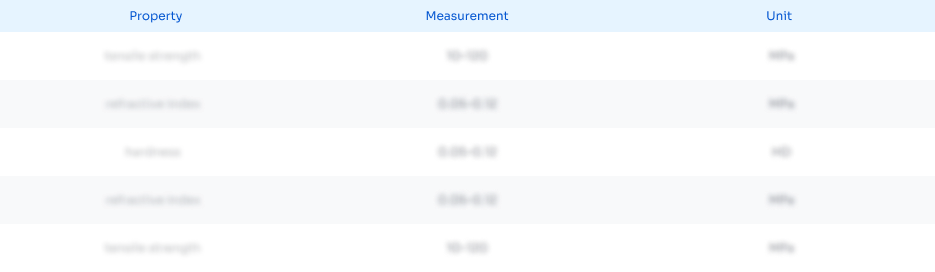
Abstract
Description
Claims
Application Information
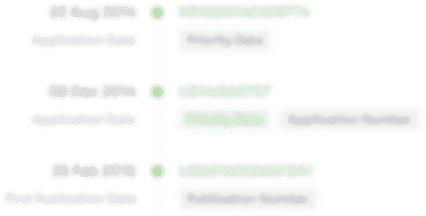
- R&D Engineer
- R&D Manager
- IP Professional
- Industry Leading Data Capabilities
- Powerful AI technology
- Patent DNA Extraction
Browse by: Latest US Patents, China's latest patents, Technical Efficacy Thesaurus, Application Domain, Technology Topic, Popular Technical Reports.
© 2024 PatSnap. All rights reserved.Legal|Privacy policy|Modern Slavery Act Transparency Statement|Sitemap|About US| Contact US: help@patsnap.com