Construction method of multi-plane coding point cloud feature deep learning model based on pointpillars
A deep learning and construction method technology, applied in the field of computer vision, can solve problems such as empty unmanned driving scenes, uneven point clouds collected by lidar, and sparse distant points
- Summary
- Abstract
- Description
- Claims
- Application Information
AI Technical Summary
Problems solved by technology
Method used
Image
Examples
Embodiment 1
[0051] A method for constructing a multi-plane coded point cloud feature deep learning model based on pointpillars, comprising the following steps:
[0052] Step 1: Obtain a training sample, the training sample includes point cloud data containing the detection target and label information corresponding to the point cloud data, the label information is used to indicate the bounding box coordinates of the detection target in the point cloud data and the detection in the bounding box coordinates Classification label for the target.
[0053] Step 2: Use training samples to train the multi-plane encoding point cloud feature deep learning model, so that the recognition result obtained by inputting the point cloud data in the training sample into the trained multi-plane encoding point cloud feature deep learning model is the point cloud data. The location bounding box coordinates of the detection target and the existence probability of the target in the bounding box coordinates.
...
Embodiment 2
[0086] A method for point cloud data target detection using the pointpillars-based multi-plane coding point cloud feature deep learning model constructed in embodiment 1, the method is to input the collected point cloud data into the multi-plane coding point cloud feature deep learning model for calculation , the multi-plane encoding point cloud feature deep learning model finally outputs the bounding box coordinates of the detected target of the point cloud data and the probability that the detected target exists in the bounding box coordinates.
PUM
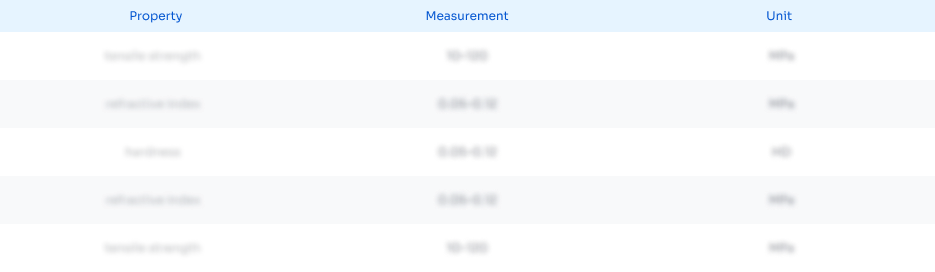
Abstract
Description
Claims
Application Information
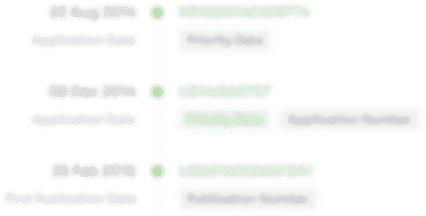
- R&D
- Intellectual Property
- Life Sciences
- Materials
- Tech Scout
- Unparalleled Data Quality
- Higher Quality Content
- 60% Fewer Hallucinations
Browse by: Latest US Patents, China's latest patents, Technical Efficacy Thesaurus, Application Domain, Technology Topic, Popular Technical Reports.
© 2025 PatSnap. All rights reserved.Legal|Privacy policy|Modern Slavery Act Transparency Statement|Sitemap|About US| Contact US: help@patsnap.com