Adaptive local low-rank matrix approximate modeling method based on recommendation system
A technology of low-rank matrix and modeling method, which is applied in the field of adaptive local low-rank matrix approximation method and approximate model, which can solve the problem of low performance of recommendation system
- Summary
- Abstract
- Description
- Claims
- Application Information
AI Technical Summary
Problems solved by technology
Method used
Image
Examples
no. 1 example
[0044] This embodiment provides a recommendation system-based adaptive local low-rank matrix approximate modeling method, the modeling method includes the following steps:
[0045] Step S1, constructing a scoring matrix.
[0046] The construction of scoring matrix described in this step is further, given a scoring matrix R=[R ij ] n×m , where the observable element R ij Records the ratings given by the i-th user to the j-th item.
[0047] In this step, usually, R is very sparse (the proportion of known values is less than 1%), so the goal is to predict the set of unknown elements Π={(i,j):R ij missing}, and denote this set as R Π . Let X=[X ij ∈{0,1}] n×m Represents an indicator matrix, if R ij is the observed data, then X ij = 1; if R ij is missing data, then X ij =0.
[0048] Step S2, generating a scoring sub-matrix according to the scoring matrix.
[0049] In order to capture the local structure from the large-scale rating matrix R, users or items are divided...
no. 2 example
[0202] This embodiment provides a recommendation system-based adaptive local low-rank matrix approximation model (AdaptiveLocal Matrix Appriximation, ALoMA), figure 1 A schematic diagram of the frame of the model is shown. Such as figure 1 As shown, the model includes:
[0203] The score matrix, user-item sub-matrix and user-item feature representation matrix obtained by the method of the first embodiment above.
[0204] further, figure 2 A schematic diagram of the structure of the model is shown. Such as figure 2 As shown, the model includes:
[0205] Rating matrix R is a matrix composed of n users and m items, c i and d j represent the categories of the i-th user and the j-th item respectively, and the ij-th scoring entry (R ij ) is assigned to the cdth submatrix in; if the i-th user belongs to the c-th category (that is, c=c i ) and the j-th item belongs to the d-th category (ie, d=d j ), then the ijth scoring entry (R ij ) will be assigned to the cdth submat...
no. 3 example
[0208] This embodiment provides a recommendation method, which uses the recommendation system-based adaptive local low-rank matrix approximation model described in the second embodiment.
PUM
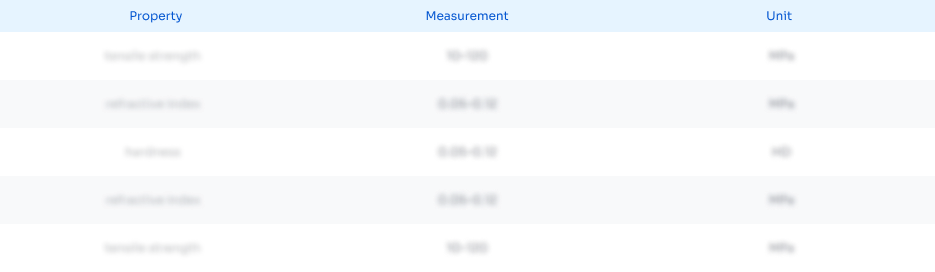
Abstract
Description
Claims
Application Information
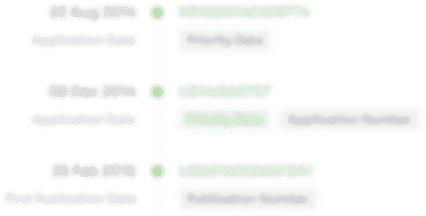
- R&D
- Intellectual Property
- Life Sciences
- Materials
- Tech Scout
- Unparalleled Data Quality
- Higher Quality Content
- 60% Fewer Hallucinations
Browse by: Latest US Patents, China's latest patents, Technical Efficacy Thesaurus, Application Domain, Technology Topic, Popular Technical Reports.
© 2025 PatSnap. All rights reserved.Legal|Privacy policy|Modern Slavery Act Transparency Statement|Sitemap|About US| Contact US: help@patsnap.com