Causal event extraction method based on self-training and noise model
A noise model and event extraction technology, applied in neural learning methods, biological neural network models, natural language data processing, etc., can solve problems such as limited effects, and achieve the effect of improving performance
- Summary
- Abstract
- Description
- Claims
- Application Information
AI Technical Summary
Problems solved by technology
Method used
Image
Examples
specific Embodiment approach 1
[0024] Specific implementation mode 1: In this implementation mode, a causal event extraction method based on self-training and noise model, the specific process is as follows:
[0025] Step 1. Collect a small amount of labeled text in the target domain, or label a small amount of unlabeled text in the target domain, and label causal event pairs. When labeling, use the labeling method of the sequence labeling task to mark a word for each word in the text a label, indicating that the word belongs to a causal event, a consequential event, or other constituents;
[0026] Step 2. First use the existing word segmentation tool to segment the marked text in step 1. Use a neural network structure, such as a pre-trained language model based on the self-attention mechanism, to calculate a word in the marked text after word segmentation. vector representation;
[0027] Step 3. Use the conditional random field model to calculate the label sequence with the highest probability from the ve...
specific Embodiment approach 2
[0036] Specific embodiment 2: The difference between this embodiment and specific embodiment 1 is that the tagging method of the sequence tagging task in the step 1 is to use BIO or BIOES and other tagging specifications, such as "money / super issue / result / got / house price / The label "B-cause / I-cause / O / O / B-effect / I-effect / I-effect / I-effect" under the BIO label specification is "B-cause / I-cause / O / O / B-effect / I-effect / I-effect / I-effect", where B-cause means the reason I-cause means the middle of the cause, B-effect means the beginning of the effect, I-effect means the middle of the result, O means other text that does not belong to the cause or effect.
[0037] Other steps and parameters are the same as those in Embodiment 1.
specific Embodiment approach 3
[0038] Specific embodiment three: the difference between this embodiment and specific embodiment one or two is: in the step two, first use the existing word segmentation tool to carry out word segmentation for the marked text in step one, and use a neural network structure, such as based on The pre-trained language model of the self-attention mechanism calculates a vector representation for the words in the labeled text after word segmentation; the specific process is:
[0039] Find the word vector corresponding to each word in the labeled text after word segmentation from the pre-trained word vector matrix, and use the word vector corresponding to each word in the labeled text after word segmentation (the word vector corresponding to each word is A row in the pre-trained vector matrix) is input into a neural network to obtain a vector representation of the fusion context information of each word;
[0040] The neural network is a recurrent neural network, a long short-term mem...
PUM
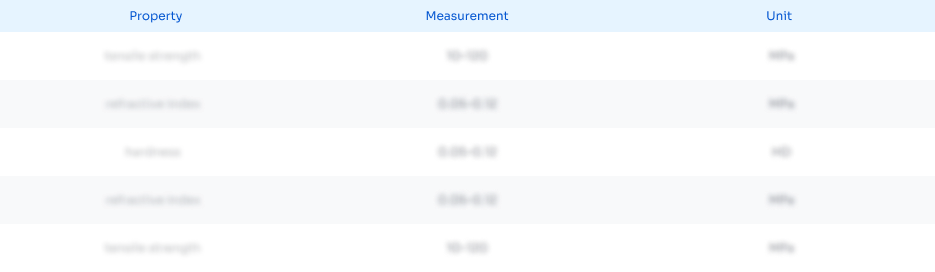
Abstract
Description
Claims
Application Information
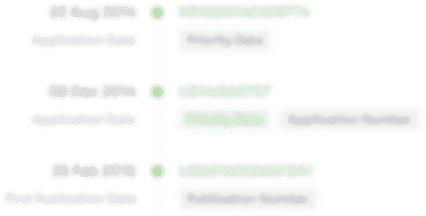
- R&D Engineer
- R&D Manager
- IP Professional
- Industry Leading Data Capabilities
- Powerful AI technology
- Patent DNA Extraction
Browse by: Latest US Patents, China's latest patents, Technical Efficacy Thesaurus, Application Domain, Technology Topic, Popular Technical Reports.
© 2024 PatSnap. All rights reserved.Legal|Privacy policy|Modern Slavery Act Transparency Statement|Sitemap|About US| Contact US: help@patsnap.com