Fly face recognition method based on deep convolutional neural network
A convolutional neural network and deep convolution technology, applied in the field of biometric identification, can solve the problems of not paying enough attention to the information difference between categories, low insect identification accuracy, and high similarity of flies, so as to reduce image gradient loss and prevent a large amount of information. The effect of losing and improving accuracy
- Summary
- Abstract
- Description
- Claims
- Application Information
AI Technical Summary
Problems solved by technology
Method used
Image
Examples
Embodiment
[0069] like figure 1 As shown, this embodiment includes the following steps:
[0070]Step 1: First, optimize the depthwise separable convolution on the basis of the MTCNN network, decompose the standard convolution in the MTCNN network into depthwise convolution and point-by-point convolution, and reduce the amount of calculation as much as possible under the premise of ensuring accuracy, so as to obtain Fly face detection box and five feature points. The MTCNN network is mainly composed of three parts: P-Net, R-Net and O-Net. The fully convolutional P-Net is used to generate candidate frames on multi-scale images to be checked, and then through R-Net and O-Net to Filtering, the total loss function formula is as follows:
[0071]
[0072] In the above formula, N is the total number of training samples, α j Indicates the weight of 0 for each loss. In P-Net and R-Net, set α det = 1, α box = 0.5, α landmark = 0.5, in O-Net, set α det = 1, α box = 0.5, α landmark =1. ...
PUM
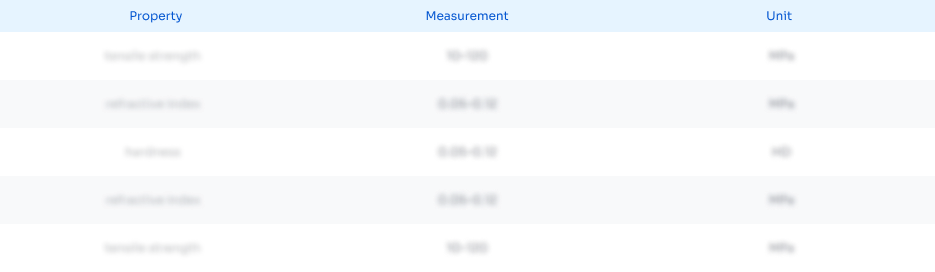
Abstract
Description
Claims
Application Information
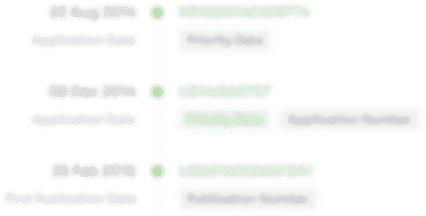
- R&D
- Intellectual Property
- Life Sciences
- Materials
- Tech Scout
- Unparalleled Data Quality
- Higher Quality Content
- 60% Fewer Hallucinations
Browse by: Latest US Patents, China's latest patents, Technical Efficacy Thesaurus, Application Domain, Technology Topic, Popular Technical Reports.
© 2025 PatSnap. All rights reserved.Legal|Privacy policy|Modern Slavery Act Transparency Statement|Sitemap|About US| Contact US: help@patsnap.com