Cloth defect detection method based on adversarial neural network, terminal and storage medium
A defect detection and neural network technology, applied in the field of cloth defect detection, can solve the problems of time-consuming and laborious, different defect shapes, and high computational complexity of deep learning, and achieve the effect of solving the difficulty of collection.
- Summary
- Abstract
- Description
- Claims
- Application Information
AI Technical Summary
Problems solved by technology
Method used
Image
Examples
Embodiment Construction
[0038] The present invention will be described in detail below in conjunction with the accompanying drawings and specific embodiments.
[0039] see figure 1 , in the present embodiment, the present invention provides a method for detecting cloth defects based on an adversarial neural network, the testing method is composed of training and testing phases, including:
[0040] Step 1: Train the GAN model according to the positive samples of the cloth, and output the GAN model after the training is completed. see figure 2 , figure 2 It is a flow chart of the training phase. By collecting positive samples of cloth, the GAN model is trained after preprocessing the positive samples, and the GAN model is output after the training is completed. The training of the GAN model adopts offline training. This detection method uses the collection of positive samples to train the model, which can avoid the problem that there are many kinds of cloth defects and are difficult to collect, and...
PUM
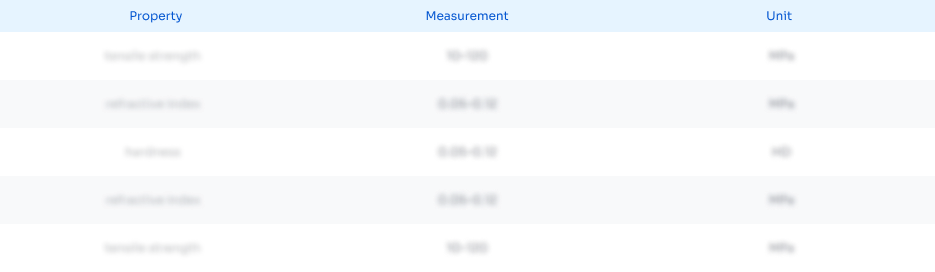
Abstract
Description
Claims
Application Information
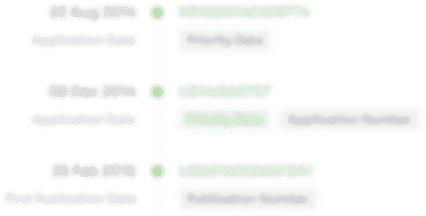
- R&D Engineer
- R&D Manager
- IP Professional
- Industry Leading Data Capabilities
- Powerful AI technology
- Patent DNA Extraction
Browse by: Latest US Patents, China's latest patents, Technical Efficacy Thesaurus, Application Domain, Technology Topic, Popular Technical Reports.
© 2024 PatSnap. All rights reserved.Legal|Privacy policy|Modern Slavery Act Transparency Statement|Sitemap|About US| Contact US: help@patsnap.com